Loading... # stable diffusion代码阅读笔记 > 本文参考的配置文件信息: > > `AutoencoderKL`:`stable-diffusion\configs\autoencoder\autoencoder_kl_32x32x4.yaml` > > `latent-diffusion`:`stable-diffusion\configs\latent-diffusion\lsun_churches-ldm-kl-8.yaml` # ldm ## modules ### diffusionmodules #### model.py ##### `Nromalize` 函数 ```python def Normalize(in_channels, num_groups=32): """创建GroupNorm层 Args: in_channels: 输入通道数 num_groups: 分组数量. Defaults to 32. Returns: 返回一个 torch.nn.GroupNorm 层的实例 """ return torch.nn.GroupNorm(num_groups=num_groups, num_channels=in_channels, eps=1e-6, affine=True) ``` 这个方法定义了一个归一化层的方式,使用群归一化有利于提高训练速度和模型稳定性 ##### `ResnetBlock`类 这个类定义了使用的残差块的模型,前向传播模型如下图所示 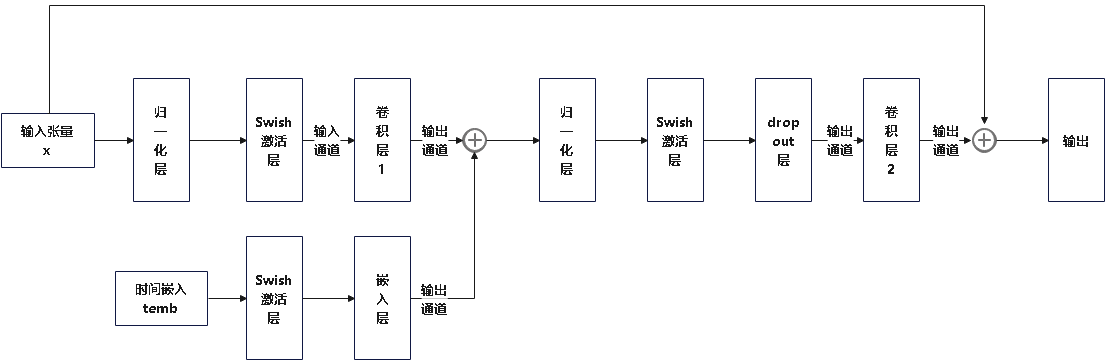 注释代码如下: ```python class ResnetBlock(nn.Module): def __init__(self, *, in_channels, out_channels=None, conv_shortcut=False, dropout, temb_channels=512): """Resnet模块实现 Args: in_channels: 输入通道数 dropout: Dropout率 out_channels: 输出通道数. Defaults to None. conv_shortcut: 是否使用卷积快速链接. Defaults to False. temb_channels: 时间嵌入通道数. Defaults to 512. """ super().__init__() self.in_channels = in_channels out_channels = in_channels if out_channels is None else out_channels self.out_channels = out_channels self.use_conv_shortcut = conv_shortcut self.norm1 = Normalize(in_channels) self.conv1 = torch.nn.Conv2d(in_channels, out_channels, kernel_size=3, stride=1, padding=1) if temb_channels > 0: self.temb_proj = torch.nn.Linear(temb_channels, out_channels) self.norm2 = Normalize(out_channels) self.dropout = torch.nn.Dropout(dropout) self.conv2 = torch.nn.Conv2d(out_channels, out_channels, kernel_size=3, stride=1, padding=1) if self.in_channels != self.out_channels: if self.use_conv_shortcut: self.conv_shortcut = torch.nn.Conv2d(in_channels, out_channels, kernel_size=3, stride=1, padding=1) else: self.nin_shortcut = torch.nn.Conv2d(in_channels, out_channels, kernel_size=1, stride=1, padding=0) def forward(self, x, temb): """前线传播方法,用于计算输入张量x经过Resnet block后的输出 Args: x: 输入张量 temb: 时间嵌入 Returns: 残差块的输出 """ h = x h = self.norm1(h) h = nonlinearity(h) h = self.conv1(h) if temb is not None: h = h + self.temb_proj(nonlinearity(temb))[:,:,None,None] # 拓展temp为四维 h = self.norm2(h) h = nonlinearity(h) h = self.dropout(h) h = self.conv2(h) if self.in_channels != self.out_channels: if self.use_conv_shortcut: x = self.conv_shortcut(x) else: x = self.nin_shortcut(x) return x+h ``` 其中定义的`norm1`和`norm2`来自`torch.nn.GroupNorm`,为一个群归一化层 ##### `AttnBlock`类 这个类定义了经典的自注意力机制,其前向传播过程的模型如下 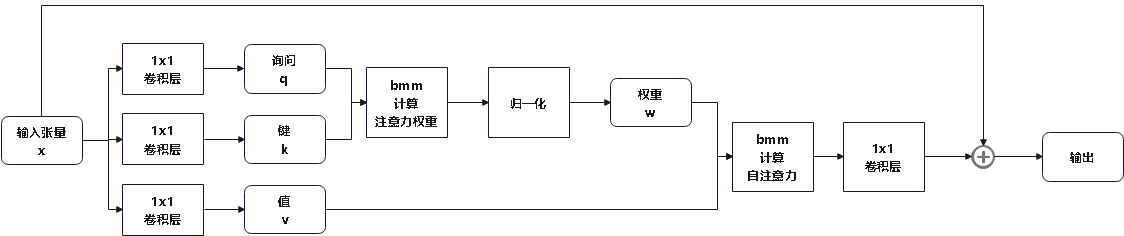 详细代码如下图所示 ```python class AttnBlock(nn.Module): def __init__(self, in_channels): """经典自注意力模块 Args: in_channels: 输入通道数 """ super().__init__() self.in_channels = in_channels self.norm = Normalize(in_channels) self.q = torch.nn.Conv2d(in_channels, in_channels, kernel_size=1, stride=1, padding=0) # 对通道层做的线性变换 self.k = torch.nn.Conv2d(in_channels, in_channels, kernel_size=1, stride=1, padding=0) self.v = torch.nn.Conv2d(in_channels, in_channels, kernel_size=1, stride=1, padding=0) self.proj_out = torch.nn.Conv2d(in_channels, in_channels, kernel_size=1, stride=1, padding=0) # 用于将经过注意力计算后的输出重新投影到输入维度的卷积层 def forward(self, x): """前向传播,计算输入x的自注意力 Args: x: 输入向量 Returns: _description_ """ h_ = x h_ = self.norm(h_) q = self.q(h_) k = self.k(h_) v = self.v(h_) # compute attention b,c,h,w = q.shape q = q.reshape(b,c,h*w) q = q.permute(0,2,1) # b,hw,c k = k.reshape(b,c,h*w) # b,c,hw w_ = torch.bmm(q,k) # b,hw,hw w[b,i,j]=sum_c q[b,i,c]k[b,c,j] 计算注意力权重,每个位置之间的关系 w_ = w_ * (int(c)**(-0.5)) # 对注意力权重进行缩放,保证数值稳定 w_ = torch.nn.functional.softmax(w_, dim=2) # attend to values v = v.reshape(b,c,h*w) w_ = w_.permute(0,2,1) # b,hw,hw (first hw of k, second of q) h_ = torch.bmm(v,w_) # b, c,hw (hw of q) h_[b,c,j] = sum_i v[b,c,i] w_[b,i,j] h_ = h_.reshape(b,c,h,w) h_ = self.proj_out(h_) return x+h_ ``` ##### `LinearAttension`类 LinearAttension类实现了一个优化过的自注意力算法,具体而言他通过改变矩阵的计算次序,将时间复杂度从$O(N^2)$降低到$O(N)$ 详细代码如下: ```python class LinearAttention(nn.Module): def __init__(self, dim, heads=4, dim_head=32): """实现了一个线性注意力机制加速注意力计算,实现方式与AttnBlock类似,但比AttnBlock快\n 先计算v和softmax(k)的乘积在与q乘法,复杂度从O(N^2)到O(N) Args: dim: 输入特征维度 heads: 注意力头数量. Defaults to 4. dim_head: 每个注意力头维度. Defaults to 32. """ super().__init__() self.heads = heads hidden_dim = dim_head * heads # 隐藏层维度 self.to_qkv = nn.Conv2d(dim, hidden_dim * 3, 1, bias = False) #1x1卷积层: q, k, v每个向量的维度都是hidden_dim self.to_out = nn.Conv2d(hidden_dim, dim, 1) # 1x1卷积层:重新投影回初始维度 def forward(self, x): b, c, h, w = x.shape qkv = self.to_qkv(x) q, k, v = rearrange(qkv, 'b (qkv heads c) h w -> qkv b heads c (h w)', heads = self.heads, qkv=3) k = k.softmax(dim=-1) context = torch.einsum('bhdn,bhen->bhde', k, v) out = torch.einsum('bhde,bhdn->bhen', context, q) out = rearrange(out, 'b heads c (h w) -> b (heads c) h w', heads=self.heads, h=h, w=w) return self.to_out(out) ``` ##### `LinAttnBlock`类 `LinAttnBlock`类继承自`LinearAttension`,设定了输入特征数为输入通道数,注意力头的数量为1 详细代码: ```python class LinAttnBlock(LinearAttention): """to match AttnBlock usage""" def __init__(self, in_channels): """继承自LinearAttention,是一个注意力头为1个的特殊的线性注意力机制 Args: in_channels: 输入通道数 """ super().__init__(dim=in_channels, heads=1, dim_head=in_channels) ``` ##### `make_attn` 函数 ```python def make_attn(in_channels, attn_type="vanilla"): """注意力模块选择函数 Args: in_channels: 输入通道数 attn_type: 注意力模块. Defaults to "vanilla". Returns: 返回所选择的注意力模块实例 """ assert attn_type in ["vanilla", "linear", "none"], f'attn_type {attn_type} unknown' print(f"making attention of type '{attn_type}' with {in_channels} in_channels") if attn_type == "vanilla": return AttnBlock(in_channels) elif attn_type == "none": return nn.Identity(in_channels) # 输入是什么输出就是什么 else: return LinAttnBlock(in_channels) ``` `make_attn `函数指定了注意力模块的种类,根据`attn_type`的不同取值提供了如下三种注意力模块 - `vanilla`: 经典自注意力模块,详见`AttnBlock类` - `linear`: 优化的自注意力模块,时间复杂度降低到O(N),详见`LinearAttension`类 - `none`: 线性层,即什么也不做,输入是什么,输出就是什么 ##### `Downsample` 类 Downsample类实现了图像的下采样操作,他提供了两种图像下采样方法 - 平均池化 - 卷积 通过`with_conv`来判断使用哪一种下采样方式实现下采样 注释代码如下: ```python class Downsample(nn.Module): def __init__(self, in_channels, with_conv): """图像下采样模块 Args: in_channels: 输入通道数 with_conv: 是否使用卷积下采样 """ super().__init__() self.with_conv = with_conv if self.with_conv: # no asymmetric padding in torch conv, must do it ourselves self.conv = torch.nn.Conv2d(in_channels, in_channels, kernel_size=3, stride=2, padding=0) # 使用卷积层将图像尺寸减小为原来的一半 def forward(self, x): if self.with_conv: pad = (0,1,0,1) # 手动进行非对称填充,右面和底面填充1个像素 x = torch.nn.functional.pad(x, pad, mode="constant", value=0) x = self.conv(x) else: x = torch.nn.functional.avg_pool2d(x, kernel_size=2, stride=2) # 平均池化,张量尺寸减半 return x ``` ##### `Encoder` 类 Encoder类实现了对于源输入的编码过程,从模型结构上来说使用的是`Unet`结构的下采样和中间层部分. 模型的前向传播过程如图所示: 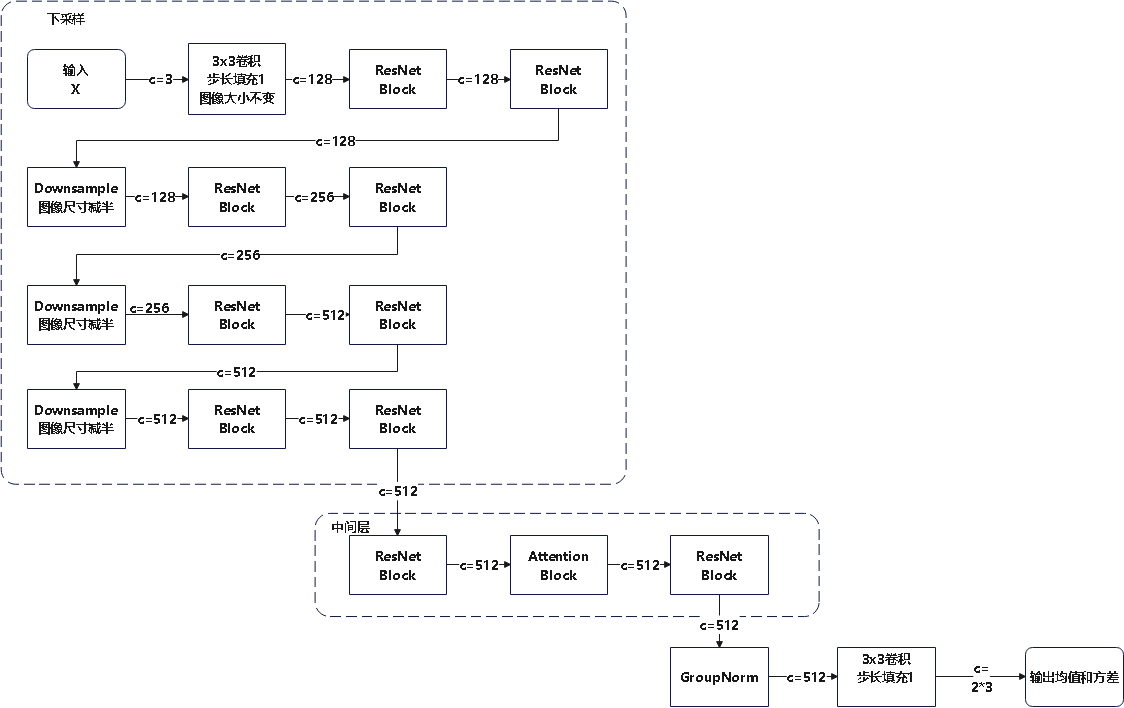 根据模型的配置文件参数,`AutoEncoderKL`在下采样过程中没有用到`AttnBlock`,他的目的是将输入图像编码为潜在变量Z的分布的描述,包括均值和方差. Encoder类代码如下: ```python class Encoder(nn.Module): def __init__(self, *, ch, out_ch, ch_mult=(1,2,4,8), num_res_blocks, attn_resolutions, dropout=0.0, resamp_with_conv=True, in_channels, resolution, z_channels, double_z=True, use_linear_attn=False, attn_type="vanilla", **ignore_kwargs): """为AutoEncoderKL的编码器部分 Args: ch: 初始通道数,用于第一层卷积 out_ch: 最终输出的通道数 num_res_blocks: 每个分辨率层中的残差块数量 attn_resolutions: 在哪些分辨率下使用注意力机制 in_channels: 输入图像的通道数 resolution: 输入图像的分辨率 z_channels: 最终潜在空间的维度数 ch_mult: 通道数的倍增系数,每一层的通道数是初始通道数乘以一个倍增系数. Defaults to (1,2,4,8). dropout: 用于控制ResnetBlock中的丢弃率. Defaults to 0.0. resamp_with_conv: 下采样时是否使用卷积操作. Defaults to True. double_z: 控制输出的通道数是否加倍,用于生成均值和标准差. Defaults to True. use_linear_attn: 是否使用线性注意力代替标准注意力. Defaults to False. attn_type: 使用的注意力类型. Defaults to "vanilla". """ super().__init__() if use_linear_attn: attn_type = "linear" self.ch = ch self.temb_ch = 0 # 时间嵌入的通道数 self.num_resolutions = len(ch_mult) self.num_res_blocks = num_res_blocks self.resolution = resolution self.in_channels = in_channels # downsampling self.conv_in = torch.nn.Conv2d(in_channels, self.ch, kernel_size=3, stride=1, padding=1) # 图像大小保持不变 curr_res = resolution in_ch_mult = (1,)+tuple(ch_mult) # (1, 1, 2, 4, 8) self.in_ch_mult = in_ch_mult self.down = nn.ModuleList() for i_level in range(self.num_resolutions): # i_level初值为1 block = nn.ModuleList() attn = nn.ModuleList() block_in = ch*in_ch_mult[i_level] block_out = ch*ch_mult[i_level] for i_block in range(self.num_res_blocks): block.append(ResnetBlock(in_channels=block_in, out_channels=block_out, temb_channels=self.temb_ch, dropout=dropout)) block_in = block_out if curr_res in attn_resolutions: attn.append(make_attn(block_in, attn_type=attn_type)) down = nn.Module() down.block = block down.attn = attn if i_level != self.num_resolutions-1: down.downsample = Downsample(block_in, resamp_with_conv) curr_res = curr_res // 2 self.down.append(down) # middle self.mid = nn.Module() self.mid.block_1 = ResnetBlock(in_channels=block_in, out_channels=block_in, temb_channels=self.temb_ch, dropout=dropout) self.mid.attn_1 = make_attn(block_in, attn_type=attn_type) self.mid.block_2 = ResnetBlock(in_channels=block_in, out_channels=block_in, temb_channels=self.temb_ch, dropout=dropout) # end self.norm_out = Normalize(block_in) self.conv_out = torch.nn.Conv2d(block_in, 2*z_channels if double_z else z_channels, kernel_size=3, stride=1, padding=1) def forward(self, x): """前向传播方法,经过下采样和中间层得到潜在变量z Args: x: 输入特征图 Returns: 潜在变量z,维度为z_channels或2*z_channels,包括均值和方差 """ # timestep embedding temb = None # downsampling hs = [self.conv_in(x)] for i_level in range(self.num_resolutions): for i_block in range(self.num_res_blocks): h = self.down[i_level].block[i_block](hs[-1], temb) if len(self.down[i_level].attn) > 0: h = self.down[i_level].attn[i_block](h) hs.append(h) if i_level != self.num_resolutions-1: hs.append(self.down[i_level].downsample(hs[-1])) # middle h = hs[-1] h = self.mid.block_1(h, temb) h = self.mid.attn_1(h) h = self.mid.block_2(h, temb) # end h = self.norm_out(h) h = nonlinearity(h) h = self.conv_out(h) return h ``` ##### `Decoder`类 Decoder类实现了对于潜在变量z的解码,将潜在变量z解码为生成图像h,从模型上来说使用的是Unet的右半部和上采样部分 模型的前向传播过程如图所示: 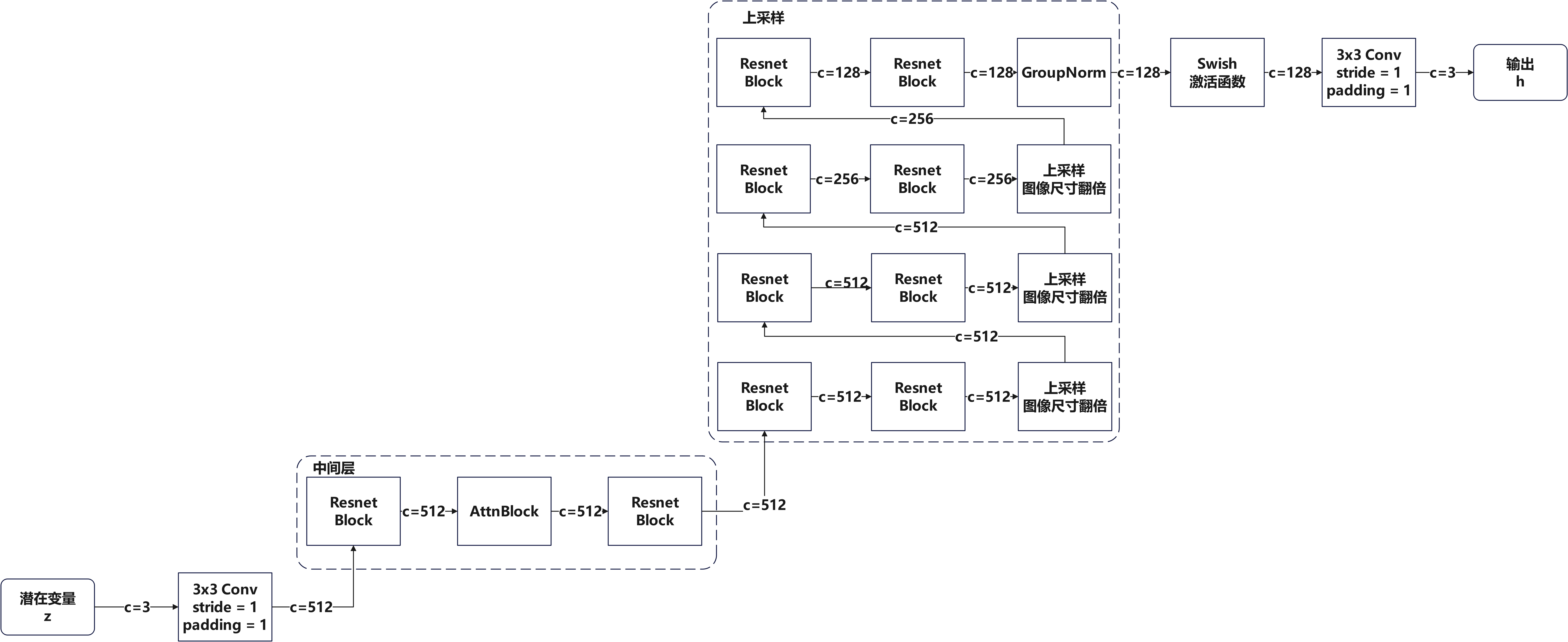 Decoder类代码如下: ```python class Decoder(nn.Module): def __init__(self, *, ch, out_ch, ch_mult=(1,2,4,8), num_res_blocks, attn_resolutions, dropout=0.0, resamp_with_conv=True, in_channels, resolution, z_channels, give_pre_end=False, tanh_out=False, use_linear_attn=False, attn_type="vanilla", **ignorekwargs): """解码器,将潜在变量z转换为生成图像 Args: ch: 初始通道数,控制网络中的通道数 out_ch: 最终输出的通道数 num_res_blocks: 每一层中 Resnet Block 的数量 attn_resolutions: 决定在哪些分辨率层应用注意力机制 in_channels: 输入通道数 resolution: 原始输入的分辨率 z_channels: 潜在空间的通道数,即编码后的特征图大小 ch_mult: 通道倍增系数,用于控制每层的通道数变化. Defaults to (1,2,4,8). dropout: Dropout 的概率. Defaults to 0.0. resamp_with_conv: 是否使用卷积进行上采样. Defaults to True. give_pre_end: 如果为 True, 返回最终卷积之前的特征图. Defaults to False. tanh_out: 如果为 True, 使用 tanh 函数将输出值范围限制在 [-1, 1]. Defaults to False. use_linear_attn: 是否使用线性注意力. Defaults to False. attn_type: 注意力类型. Defaults to "vanilla". """ super().__init__() if use_linear_attn: attn_type = "linear" self.ch = ch self.temb_ch = 0 self.num_resolutions = len(ch_mult) self.num_res_blocks = num_res_blocks self.resolution = resolution self.in_channels = in_channels self.give_pre_end = give_pre_end self.tanh_out = tanh_out # compute in_ch_mult, block_in and curr_res at lowest res in_ch_mult = (1,)+tuple(ch_mult) # (1, 1, 2, 4, 8) block_in = ch*ch_mult[self.num_resolutions-1] curr_res = resolution // 2**(self.num_resolutions-1) self.z_shape = (1,z_channels,curr_res,curr_res) print("Working with z of shape {} = {} dimensions.".format( self.z_shape, np.prod(self.z_shape))) # z to block_in self.conv_in = torch.nn.Conv2d(z_channels, block_in, kernel_size=3, stride=1, padding=1) # middle self.mid = nn.Module() self.mid.block_1 = ResnetBlock(in_channels=block_in, out_channels=block_in, temb_channels=self.temb_ch, dropout=dropout) self.mid.attn_1 = make_attn(block_in, attn_type=attn_type) self.mid.block_2 = ResnetBlock(in_channels=block_in, out_channels=block_in, temb_channels=self.temb_ch, dropout=dropout) # upsampling self.up = nn.ModuleList() for i_level in reversed(range(self.num_resolutions)): block = nn.ModuleList() attn = nn.ModuleList() block_out = ch*ch_mult[i_level] for i_block in range(self.num_res_blocks+1): block.append(ResnetBlock(in_channels=block_in, out_channels=block_out, temb_channels=self.temb_ch, dropout=dropout)) block_in = block_out if curr_res in attn_resolutions: attn.append(make_attn(block_in, attn_type=attn_type)) up = nn.Module() up.block = block up.attn = attn if i_level != 0: up.upsample = Upsample(block_in, resamp_with_conv) curr_res = curr_res * 2 self.up.insert(0, up) # 将up插入到self.up列表的开头 # end self.norm_out = Normalize(block_in) self.conv_out = torch.nn.Conv2d(block_in, out_ch, kernel_size=3, stride=1, padding=1) def forward(self, z): """前向传播方法,从最初的潜在变量z解码得到生成图像 Args: z: 潜在变量z Returns: 解码得到的生成图像 """ #assert z.shape[1:] == self.z_shape[1:] self.last_z_shape = z.shape # timestep embedding temb = None # z to block_in h = self.conv_in(z) # middle h = self.mid.block_1(h, temb) h = self.mid.attn_1(h) h = self.mid.block_2(h, temb) # upsampling for i_level in reversed(range(self.num_resolutions)): for i_block in range(self.num_res_blocks+1): h = self.up[i_level].block[i_block](h, temb) if len(self.up[i_level].attn) > 0: h = self.up[i_level].attn[i_block](h) if i_level != 0: h = self.up[i_level].upsample(h) # end if self.give_pre_end: return h h = self.norm_out(h) h = nonlinearity(h) h = self.conv_out(h) if self.tanh_out: h = torch.tanh(h) return h ``` ### distributions #### distributions.py ##### `DiagonalGaussianDistribution`类 对角高斯分布类使用编码器`Encoder`对输入特征`x`的编码得到的潜在变量`z`,根据`z`中含有的均值方差等信息建立了对角高斯分布,提供了计算均值方差、采样、计算KL散度、计算负对数似然等方法 ###### `__init__`方法 ```python def __init__(self, parameters, deterministic=False): """对角高斯分布,存储对角高斯分布的均值方差等参数,并提供了采样方式 Args: parameters: 潜在变量z deterministic: 参数是否为确定性分布. Defaults to False. """ self.parameters = parameters self.mean, self.logvar = torch.chunk(parameters, 2, dim=1) self.logvar = torch.clamp(self.logvar, -30.0, 20.0) # 防止方差过大过小 self.deterministic = deterministic self.std = torch.exp(0.5 * self.logvar) # 标准差 self.var = torch.exp(self.logvar) # 方差 if self.deterministic: self.var = self.std = torch.zeros_like(self.mean).to(device=self.parameters.device) # 确定性分布方差为0 ``` 构造函数中根据潜在变量`z`确定了对角高斯分布的均值和方差信息,如果`deterministic`为真,则使方差为0,让高斯分布退化为一个确定的分布 ###### `sample`方法 ```python def sample(self): """从对角高斯分布中采样\n x=μ+σ⋅ϵ\nϵ为高斯白噪声 Returns: 返回采样得到的变量 """ x = self.mean + self.std * torch.randn(self.mean.shape).to(device=self.parameters.device) return x ``` `sample`方法使用如下公式计算采样得到的分布 $$ x=\mu+\sigma\epsilon,\quad\epsilon \sim N(0, I) $$ ###### `kl` 方法 ```python def kl(self, other=None): """计算KL散度 Args: other: 与哪一个分布计算KL散度,默认与正态分布计算. Defaults to None. Returns: _description_ """ if self.deterministic: return torch.Tensor([0.]) else: if other is None: return 0.5 * torch.sum(torch.pow(self.mean, 2) + self.var - 1.0 - self.logvar, dim=[1, 2, 3]) else: return 0.5 * torch.sum( torch.pow(self.mean - other.mean, 2) / other.var + self.var / other.var - 1.0 - self.logvar + other.logvar, dim=[1, 2, 3]) ``` - **KL 散度** 用于衡量两个分布之间的差异 当 `other` 为 `None` 时,表示计算与标准正态分布(均值为 0,方差为 1)的 KL 散度,公式如下: $$ D_{KL}(q || p) = 0.5 \cdot \sum \left( \mu^2 + \sigma^2 - 1 - \log(\sigma^2) \right) $$ - 当 `other` 不为 `None` 时,表示计算与另一个对角高斯分布的 KL 散度: $$ D_{KL}(q || p) = 0.5 \cdot \sum \left( \frac{(\mu_q - \mu_p)^2}{\sigma_p^2} + \frac{\sigma_q^2}{\sigma_p^2} - 1 - \log \frac{\sigma_q^2}{\sigma_p^2} \right) $$ ###### `nll` 方法 ```python def nll(self, sample, dims=[1,2,3]): """计算负对数似然 Args: sample: 真实样本 dims: 维度信息. Defaults to [1,2,3]. Returns: _description_ """ if self.deterministic: return torch.Tensor([0.]) logtwopi = np.log(2.0 * np.pi) return 0.5 * torch.sum( logtwopi + self.logvar + torch.pow(sample - self.mean, 2) / self.var, dim=dims) ``` - **负对数似然(NLL)** 是一种衡量数据点与分布拟合程度的指标。公式为: $$ \text{NLL} = 0.5 \cdot \sum \left( \log(2\pi) + \log(\sigma^2) + \frac{(x - \mu)^2}{\sigma^2} \right) $$ 其中,$x$ 是真实样本,$\mu$ 是均值,$\sigma^2$ 是方差。 $$ ###### `mode` 方法 ```python def mode(self): """众数,高斯分布的众数即均值 Returns: 返回高斯分布的众数(均值) """ return self.mean ``` - **众数**(`mode`)即分布的均值,因为高斯分布的众数就是其均值。 ### discriminator #### model.py ##### `NLayerDiscriminator`类 这个函数实现了一个GAN判别器,用于判断输入图像的局部区域是否是真是图像,模型通过不同通道数的卷积和激活函数提取生成图像并判别真假,模型结构如下: 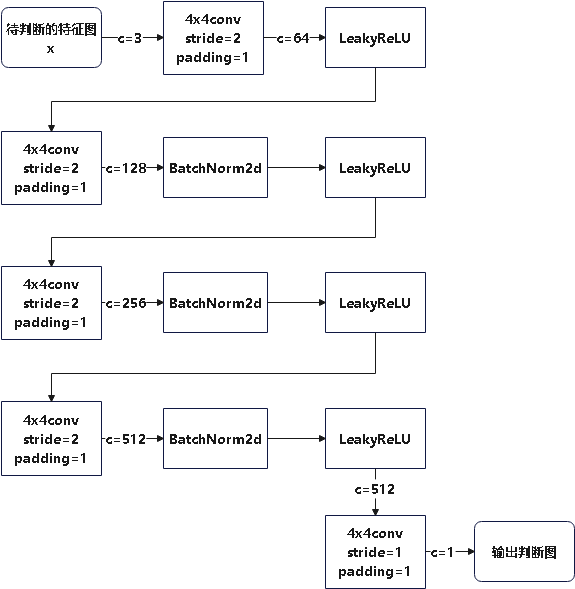 详细注释代码如下: ```python class NLayerDiscriminator(nn.Module): """Defines a PatchGAN discriminator as in Pix2Pix --> see https://github.com/junyanz/pytorch-CycleGAN-and-pix2pix/blob/master/models/networks.py """ def __init__(self, input_nc=3, ndf=64, n_layers=3, use_actnorm=False): """PatchGAN 判别器,用于判断输入图像的局部区域是否为真实图像。它通过多层卷积逐步提取特征,并输出一个单通道的特征图,表示每个局部区域的真实性。这个结构中的层数和滤波器数量可以根据需求调整。 Args: input_nc: 输入图像的通道数. Defaults to 3. ndf: 第一层卷积层的输出通道数. Defaults to 64. n_layers: 卷积层的层数. Defaults to 3. use_actnorm: 是否使用激活归一化层. Defaults to False. """ super(NLayerDiscriminator, self).__init__() if not use_actnorm: norm_layer = nn.BatchNorm2d else: norm_layer = ActNorm if type(norm_layer) == functools.partial: # BatchNorm2d 自带仿射变换(即有偏置和缩放参数) use_bias = norm_layer.func != nn.BatchNorm2d else: use_bias = norm_layer != nn.BatchNorm2d kw = 4 # 卷积核大小 padw = 1 # 填充大小 sequence = [nn.Conv2d(input_nc, ndf, kernel_size=kw, stride=2, padding=padw), nn.LeakyReLU(0.2, True)] nf_mult = 1 nf_mult_prev = 1 for n in range(1, n_layers): # gradually increase the number of filters nf_mult_prev = nf_mult nf_mult = min(2 ** n, 8) sequence += [ nn.Conv2d(ndf * nf_mult_prev, ndf * nf_mult, kernel_size=kw, stride=2, padding=padw, bias=use_bias), norm_layer(ndf * nf_mult), nn.LeakyReLU(0.2, True) ] nf_mult_prev = nf_mult nf_mult = min(2 ** n_layers, 8) sequence += [ nn.Conv2d(ndf * nf_mult_prev, ndf * nf_mult, kernel_size=kw, stride=1, padding=padw, bias=use_bias), norm_layer(ndf * nf_mult), nn.LeakyReLU(0.2, True) ] sequence += [ nn.Conv2d(ndf * nf_mult, 1, kernel_size=kw, stride=1, padding=padw)] # output 1 channel prediction map self.main = nn.Sequential(*sequence) def forward(self, input): """PatchGAN 判别器,用于判断输入图像的局部区域是否为真实图像。它通过多层卷积逐步提取特征,并输出一个单通道的特征图,表示每个局部区域的真实性。这个结构中的层数和滤波器数量可以根据需求调整。 Args: input: 输入图像 Returns: 通道数为1的卷积,用于判断图像真实性 """ """Standard forward.""" return self.main(input) ``` ### losses #### vqperceptual.py ##### `hinge_d_loss`函数 - 对 **真实样本**,我们希望判别器输出的分数尽可能大于 1(越大越好),因此 `1. - logits_real` 会惩罚得分小于 1 的情况。 - 对 **生成样本**,我们希望判别器输出的分数尽可能小于 -1,`1. + logits_fake` 会惩罚得分高于 -1 的情况。 注释代码: ```python def hinge_d_loss(logits_real, logits_fake): """GAN判别器损失函数 Args: logits_real: 判别器对真实样本的输出 logits_fake: 判别器对生成样本的输出 Returns: 最终的判别器损失 """ loss_real = torch.mean(F.relu(1. - logits_real)) # 计算真实样本的损失, 希望 logits_real 尽可能大于 1 loss_fake = torch.mean(F.relu(1. + logits_fake)) # 计算生成样本的损失, 希望 logits_fake 尽可能小于 -1 d_loss = 0.5 * (loss_real + loss_fake) # 求均值 return d_loss ``` #### lpips.py ##### `vgg16`类 `VGG16`使用的是固定的预训练权重参数,通过将网络整体分为五个部分,存储每个部分的输出及其对应的标签作为前向传播的整体输出. 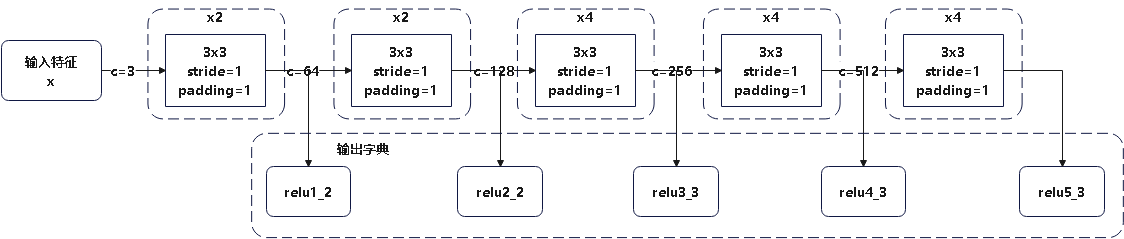 注释代码如下: ```python class vgg16(torch.nn.Module): def __init__(self, requires_grad=False, pretrained=True): """预训练的VGG16网络 Args: requires_grad: 是否需要梯度信息. Defaults to False. pretrained: 是否使用预训练权重. Defaults to True. """ super(vgg16, self).__init__() vgg_pretrained_features = models.vgg16(pretrained=pretrained).features self.slice1 = torch.nn.Sequential() self.slice2 = torch.nn.Sequential() self.slice3 = torch.nn.Sequential() self.slice4 = torch.nn.Sequential() self.slice5 = torch.nn.Sequential() self.N_slices = 5 for x in range(4): self.slice1.add_module(str(x), vgg_pretrained_features[x]) for x in range(4, 9): self.slice2.add_module(str(x), vgg_pretrained_features[x]) for x in range(9, 16): self.slice3.add_module(str(x), vgg_pretrained_features[x]) for x in range(16, 23): self.slice4.add_module(str(x), vgg_pretrained_features[x]) for x in range(23, 30): self.slice5.add_module(str(x), vgg_pretrained_features[x]) if not requires_grad: for param in self.parameters(): param.requires_grad = False def forward(self, X): """将整个网络分为五个部分,记录每个部分的输出并返回 Args: X: 输入特征x Returns: 包含网络中五个部分的输出特征的字典 """ h = self.slice1(X) h_relu1_2 = h h = self.slice2(h) h_relu2_2 = h h = self.slice3(h) h_relu3_3 = h h = self.slice4(h) h_relu4_3 = h h = self.slice5(h) h_relu5_3 = h vgg_outputs = namedtuple("VggOutputs", ['relu1_2', 'relu2_2', 'relu3_3', 'relu4_3', 'relu5_3']) out = vgg_outputs(h_relu1_2, h_relu2_2, h_relu3_3, h_relu4_3, h_relu5_3) return out ``` ##### `NetLinlayer` 类 该类实现了一个简单的1x1卷积神经网络,用于修改通道数  详细代码如下: ```python class NetLinLayer(nn.Module): def __init__(self, chn_in, chn_out=1, use_dropout=False): """通过1x1卷积层将VGG16网络的输出映射到通道数为1的特征向量 Args: chn_in: 输入通道数 chn_out: 输出通道数. Defaults to 1. use_dropout: 是否使用dropout. Defaults to False. """ super(NetLinLayer, self).__init__() layers = [nn.Dropout(), ] if (use_dropout) else [] layers += [nn.Conv2d(chn_in, chn_out, 1, stride=1, padding=0, bias=False), ] self.model = nn.Sequential(*layers) ``` ##### `ScalingLayer` 类 ```python class ScalingLayer(nn.Module): def __init__(self): """缩放层,对输入的张量标准化处理 """ super(ScalingLayer, self).__init__() self.register_buffer('shift', torch.Tensor([-.030, -.088, -.188])[None, :, None, None]) self.register_buffer('scale', torch.Tensor([.458, .448, .450])[None, :, None, None]) def forward(self, inp): """前向传播,标准化输入张量 Args: inp: 输入张量 Returns: 标准化后的张量 """ return (inp - self.shift) / self.scale ``` ##### `LPIPS` 类 `LPIPS`类计算的是两个输入图像之间的感知损失,模型如下图所示 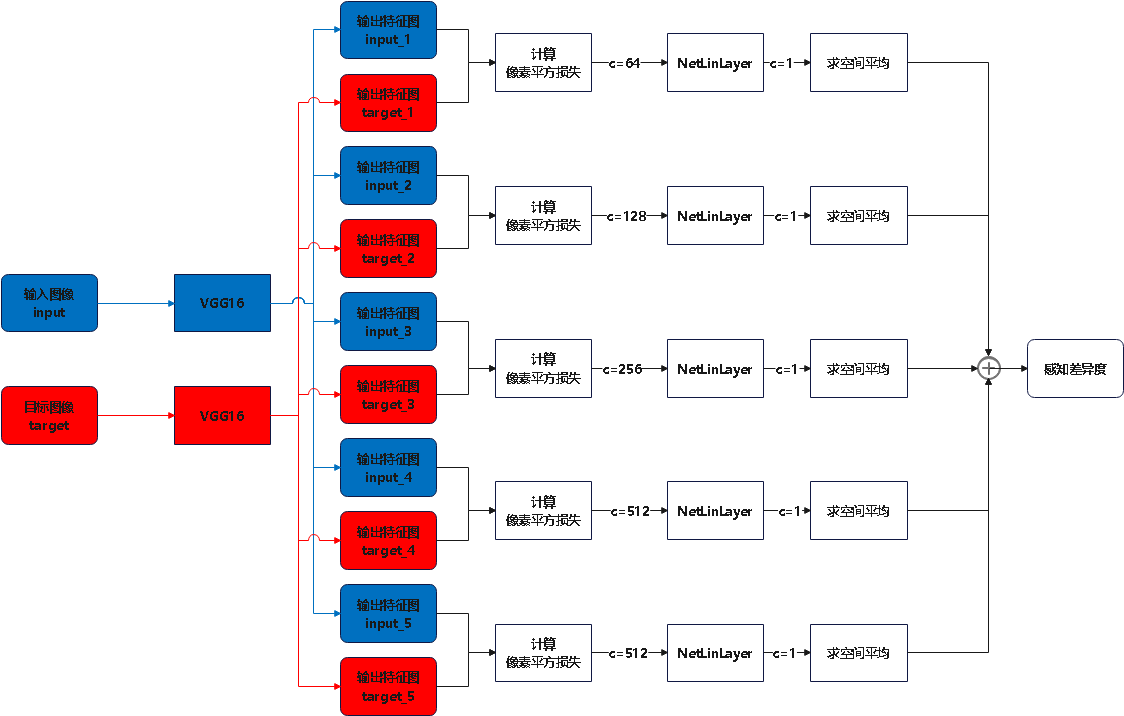 注释代码如下: ```python class LPIPS(nn.Module): # Learned perceptual metric def __init__(self, use_dropout=True): """计算感知损失,通过预训练的VGG16网络衡量两张图像之间的视觉相似性 Args: use_dropout: 用于控制是否在 NetLinLayer 中使用 dropout 层. Defaults to True. """ super().__init__() self.scaling_layer = ScalingLayer() self.chns = [64, 128, 256, 512, 512] # VGG16 网络中提取的不同特征层的通道数 self.net = vgg16(pretrained=True, requires_grad=False) # 预训练的VGG16网络 self.lin0 = NetLinLayer(self.chns[0], use_dropout=use_dropout) self.lin1 = NetLinLayer(self.chns[1], use_dropout=use_dropout) self.lin2 = NetLinLayer(self.chns[2], use_dropout=use_dropout) self.lin3 = NetLinLayer(self.chns[3], use_dropout=use_dropout) self.lin4 = NetLinLayer(self.chns[4], use_dropout=use_dropout) self.load_from_pretrained() for param in self.parameters(): param.requires_grad = False def load_from_pretrained(self, name="vgg_lpips"): ckpt = get_ckpt_path(name, "taming/modules/autoencoder/lpips") self.load_state_dict(torch.load(ckpt, map_location=torch.device("cpu")), strict=False) print("loaded pretrained LPIPS loss from {}".format(ckpt)) @classmethod def from_pretrained(cls, name="vgg_lpips"): if name != "vgg_lpips": raise NotImplementedError model = cls() ckpt = get_ckpt_path(name) model.load_state_dict(torch.load(ckpt, map_location=torch.device("cpu")), strict=False) return model def forward(self, input, target): """计算两个特征图之间的像素差异度(感知差异度) Args: input: 输入的特征图 target: 与输入特征图比较差异度的特征图 Returns: 感知差异 """ in0_input, in1_input = (self.scaling_layer(input), self.scaling_layer(target)) # 标准化缩放 outs0, outs1 = self.net(in0_input), self.net(in1_input) feats0, feats1, diffs = {}, {}, {} lins = [self.lin0, self.lin1, self.lin2, self.lin3, self.lin4] for kk in range(len(self.chns)): feats0[kk], feats1[kk] = normalize_tensor(outs0[kk]), normalize_tensor(outs1[kk]) # 标准化处理 diffs[kk] = (feats0[kk] - feats1[kk]) ** 2 # 求每个像素之间差异的平方 res = [spatial_average(lins[kk].model(diffs[kk]), keepdim=True) for kk in range(len(self.chns))] # 映射到一个通道上面去求均值[c, 1, 1] val = res[0] for l in range(1, len(self.chns)): val += res[l] # 累加获得最终的感知差异度 return val ``` #### contperceptual.py ##### `LPIPSWithDiscriminator` 类 该类用于计算并更新生成器和判别器 - 更新生成器: - 计算重构损失和感知损失 - 根据重构损失和感知损失得到负对数似然损失 - 计算KL散度(与标准正态分布) - 计算判别器损失 - 总损失函数=负对数似然损失+KL散度+判别器损失 - 更新判别器 - 计算真实图像和重建图像判别结果 - 计算对抗损失 - 总损失函数=判别器对抗损失函数 详细代码如下: ```python class LPIPSWithDiscriminator(nn.Module): def __init__(self, disc_start, logvar_init=0.0, kl_weight=1.0, pixelloss_weight=1.0, disc_num_layers=3, disc_in_channels=3, disc_factor=1.0, disc_weight=1.0, perceptual_weight=1.0, use_actnorm=False, disc_conditional=False, disc_loss="hinge"): """损失函数类,包括感知损失和判别器损失 Args: disc_start: 判别器开始工作的时间点 logvar_init: 初始化对数方差的初始值. Defaults to 0.0. kl_weight: KL散度的权重。. Defaults to 1.0. pixelloss_weight: 像素级损失的权重. Defaults to 1.0. disc_num_layers: 判别器中的层数. Defaults to 3. disc_in_channels: 判别器输入的通道数. Defaults to 3. disc_factor: 判别器的损失因子. Defaults to 1.0. disc_weight: 自适应判别器权重. Defaults to 1.0. perceptual_weight: 感知损失的权重. Defaults to 1.0. use_actnorm: 是否在判别器中使用 actnorm 层. Defaults to False. disc_conditional: 判别器是否为条件 GAN. Defaults to False. disc_loss: 判别器使用的损失函数. Defaults to "hinge". """ super().__init__() assert disc_loss in ["hinge", "vanilla"] self.kl_weight = kl_weight # 0.000001 self.pixel_weight = pixelloss_weight self.perceptual_loss = LPIPS().eval() self.perceptual_weight = perceptual_weight # output log variance self.logvar = nn.Parameter(torch.ones(size=()) * logvar_init) self.discriminator = NLayerDiscriminator(input_nc=disc_in_channels, n_layers=disc_num_layers, use_actnorm=use_actnorm ).apply(weights_init) self.discriminator_iter_start = disc_start # 50001 self.disc_loss = hinge_d_loss if disc_loss == "hinge" else vanilla_d_loss self.disc_factor = disc_factor self.discriminator_weight = disc_weight # 0.5 self.disc_conditional = disc_conditional def calculate_adaptive_weight(self, nll_loss, g_loss, last_layer=None): if last_layer is not None: nll_grads = torch.autograd.grad(nll_loss, last_layer, retain_graph=True)[0] g_grads = torch.autograd.grad(g_loss, last_layer, retain_graph=True)[0] else: nll_grads = torch.autograd.grad(nll_loss, self.last_layer[0], retain_graph=True)[0] g_grads = torch.autograd.grad(g_loss, self.last_layer[0], retain_graph=True)[0] d_weight = torch.norm(nll_grads) / (torch.norm(g_grads) + 1e-4) d_weight = torch.clamp(d_weight, 0.0, 1e4).detach() d_weight = d_weight * self.discriminator_weight return d_weight def forward(self, inputs, reconstructions, posteriors, optimizer_idx, global_step, last_layer=None, cond=None, split="train", weights=None): """AutoEncoderKL参数损失函数计算 Args: inputs: 原始输入图像 reconstructions: 模型重建的图像 posteriors: 用于计算 KL 散度的后验分布 optimizer_idx: 用于区分是在更新生成器(=1)还是判别器(=0) global_step: 当前的训练步数 last_layer: 用于自适应权重计算的最后一层. Defaults to None. cond: 条件 GAN 的输入,如果存在,生成器和判别器都会将其作为输入的一部分. Defaults to None. split: 训练模式. Defaults to "train". weights: 用于加权重建损失. Defaults to None. Returns: 最终的损失函数,日志文件 """ rec_loss = torch.abs(inputs.contiguous() - reconstructions.contiguous()) # 重构损失:inputs和resconstructions之差的绝对值 if self.perceptual_weight > 0: p_loss = self.perceptual_loss(inputs.contiguous(), reconstructions.contiguous()) # 计算感知损失,通过VGG网络计算 rec_loss = rec_loss + self.perceptual_weight * p_loss # 更新损失为重构损失+感知损失 nll_loss = rec_loss / torch.exp(self.logvar) + self.logvar # 负对数似然损失 weighted_nll_loss = nll_loss if weights is not None: weighted_nll_loss = weights*nll_loss weighted_nll_loss = torch.sum(weighted_nll_loss) / weighted_nll_loss.shape[0] # 计算每个样本平均的负对数似然损失 nll_loss = torch.sum(nll_loss) / nll_loss.shape[0] kl_loss = posteriors.kl() # 计算KL损失 kl_loss = torch.sum(kl_loss) / kl_loss.shape[0] # now the GAN part if optimizer_idx == 0: # 生成器更新 if cond is None: assert not self.disc_conditional logits_fake = self.discriminator(reconstructions.contiguous()) # 计算判别器对于重建图像的预测 else: assert self.disc_conditional logits_fake = self.discriminator(torch.cat((reconstructions.contiguous(), cond), dim=1)) g_loss = -torch.mean(logits_fake) # 反转损失函数, 优化最小化g_loss等价于最大化判别器对于重建图像的预测,即最大化判别器认为重建图像真实性 if self.disc_factor > 0.0: try: d_weight = self.calculate_adaptive_weight(nll_loss, g_loss, last_layer=last_layer) # 计算自适应权重 except RuntimeError: assert not self.training d_weight = torch.tensor(0.0) else: d_weight = torch.tensor(0.0) disc_factor = adopt_weight(self.disc_factor, global_step, threshold=self.discriminator_iter_start) # 根据时间步判断是否使用判别器损失 loss = weighted_nll_loss + self.kl_weight * kl_loss + d_weight * disc_factor * g_loss # 加权后的重建损失+加权后的KL散度+加权后的判别器损失 log = {"{}/total_loss".format(split): loss.clone().detach().mean(), "{}/logvar".format(split): self.logvar.detach(), "{}/kl_loss".format(split): kl_loss.detach().mean(), "{}/nll_loss".format(split): nll_loss.detach().mean(), "{}/rec_loss".format(split): rec_loss.detach().mean(), "{}/d_weight".format(split): d_weight.detach(), "{}/disc_factor".format(split): torch.tensor(disc_factor), "{}/g_loss".format(split): g_loss.detach().mean(), } return loss, log if optimizer_idx == 1: # 判别器更新 if cond is None: logits_real = self.discriminator(inputs.contiguous().detach()) # 计算真实图像损失 logits_fake = self.discriminator(reconstructions.contiguous().detach()) # 计算重建图像损失 else: logits_real = self.discriminator(torch.cat((inputs.contiguous().detach(), cond), dim=1)) logits_fake = self.discriminator(torch.cat((reconstructions.contiguous().detach(), cond), dim=1)) disc_factor = adopt_weight(self.disc_factor, global_step, threshold=self.discriminator_iter_start) # 判断是否计算判别器损失 d_loss = disc_factor * self.disc_loss(logits_real, logits_fake) # 计算对抗损失 log = {"{}/disc_loss".format(split): d_loss.clone().detach().mean(), "{}/logits_real".format(split): logits_real.detach().mean(), "{}/logits_fake".format(split): logits_fake.detach().mean() } return d_loss, log ``` ## models ### autoencoder.py #### `AutoencoderKL` 类 这个类实现的是第一阶段的训练任务 ##### `encode`方法 ```python def encode(self, x): """编码器 Args: x: 输入的特征图 Returns: 先验分布 """ h = self.encoder(x) # 潜在变量z moments = self.quant_conv(h) # 嵌入向量 posterior = DiagonalGaussianDistribution(moments) # 实例化为对角高斯分布作为先验分布 return posterior ``` 该函数将输入特征图转变为潜在变量z后经过嵌入层,最终实例化为对角高斯分布 模型结构如下:  ##### `decode`方法 ```python def decode(self, z): """解码器 Args: z: 采样得到的嵌入向量 Returns: 解码得到的输出特征图 """ z = self.post_quant_conv(z) dec = self.decoder(z) return dec ``` 解码器则是将嵌入层变量`z`先通过卷积映射到潜在变量`z`的维度上,然后使用解码器进行解码得到目的特征图 模型结构如下:  ##### `forward`方法 ```python def forward(self, input, sample_posterior=True): """前向传播方法,计算输入特征图经过encoder得到的先验分布,并从中采样经过解码器解码得到输出图像 Args: input: 输入特征图 sample_posterior: 是否使用采样. Defaults to True. Returns: 解码得到的图片和先验分布 """ posterior = self.encode(input) if sample_posterior: z = posterior.sample() else: z = posterior.mode() dec = self.decode(z) return dec, posterior ``` 模型如下图所示 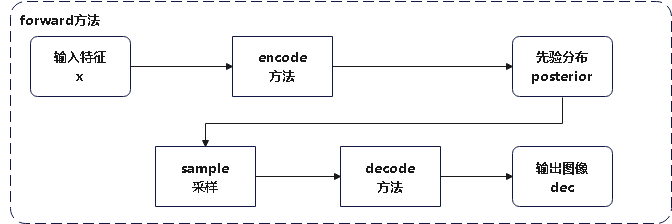 ### ddpm.py #### `DiffusionWrapper` 类 这个类实现了一个包装器,通过处理不同情况的条件输入,将条件输入和输入图像一同送进模型 注释代码如下: ```python class DiffusionWrapper(pl.LightningModule): def __init__(self, diff_model_config, conditioning_key): """一个用于扩散模型的包装器,提供了一种灵活的方式来处理不同的条件输入类型 Args: diff_model_config: 一个配置字典,用于创建扩散模型的配置 conditioning_key: 决定如何将条件信息与扩散模型结合 """ super().__init__() self.diffusion_model = instantiate_from_config(diff_model_config) self.conditioning_key = conditioning_key assert self.conditioning_key in [None, 'concat', 'crossattn', 'hybrid', 'adm'] def forward(self, x, t, c_concat: list = None, c_crossattn: list = None): """处理条件输入,将条件输入和输入图像结合,并通过模型 Args: x: 输入图像或噪声 t: 扩散过程中的时间步数 c_concat: 在 concat 和 hybrid 模式下使用,条件信息会与输入图像拼接在一起. Defaults to None. c_crossattn: 在 crossattn、hybrid 和 adm 模式下使用,作为上下文传递给模型. Defaults to None. Raises: NotImplementedError: _description_ Returns: _description_ """ if self.conditioning_key is None: out = self.diffusion_model(x, t) # 直接将输入图像和时间步数传给扩散模型,不使用条件信息 elif self.conditioning_key == 'concat': xc = torch.cat([x] + c_concat, dim=1) # 将输入图像 x 和条件信息 c_concat 拼接在一起,然后传给扩散模型 out = self.diffusion_model(xc, t) elif self.conditioning_key == 'crossattn': cc = torch.cat(c_crossattn, 1) # 将条件信息 c_crossattn 拼接在一起,作为上下文信息传给扩散模型 out = self.diffusion_model(x, t, context=cc) elif self.conditioning_key == 'hybrid': # 同时使用拼接和上下文信息,输入图像和条件信息 c_concat 拼接后传给模型,同时将条件信息 c_crossattn 作为上下文传递 xc = torch.cat([x] + c_concat, dim=1) cc = torch.cat(c_crossattn, 1) out = self.diffusion_model(xc, t, context=cc) elif self.conditioning_key == 'adm': # 使用 ADM 特定的条件信息,将 c_crossattn[0] 作为 y 传给模型 cc = c_crossattn[0] out = self.diffusion_model(x, t, y=cc) else: raise NotImplementedError() return out ``` #### `ddpm` 类 ##### DDPM前向过程 $$ \begin{eqnarray} x_t&=&\sqrt{\alpha_t}x_{t-1}+\sqrt{1-\alpha_t}\bar{\epsilon_t},\quad \mathcal{N}\sim (0, I), \quad \beta_t=1-\alpha_t\\ x_t&=&\sqrt{\bar{\alpha_t}}x_{0}+\sqrt{1-\bar{\alpha_t}}\bar{\epsilon_t},\quad \mathcal{N}\sim (0, I),\quad \bar{\alpha_t}=\alpha_t\alpha_{t-1}...\alpha_1 \end{eqnarray} $$ 据此可以用重参数化技巧写成: $$ x_t \sim p(x_t\mid x_{t-1})=\mathcal{N}(x_t; \sqrt{\alpha_t}x_{t-1}, (1-\alpha_t)I)\\ x_t \sim p(x_t\mid x_{0})=\mathcal{N}(x_t; \sqrt{\bar{\alpha_t}}x_{0}, (1-\bar{\alpha_t})I)\\ $$ ##### DDPM反向过程 根据贝叶斯定理有 $$ p(x_{t-1}\mid x_t)=\frac{p(x_t\mid x_{t-1})p(x_{t-1})}{p(x_t)} $$ 可以在给定$x_0$条件下使用贝叶斯定理: $$ p(x_{t-1}\mid x_t, x_0)=\frac{p(x_t\mid x_{t-1}, x_0)p(x_{t-1} \mid x_0)}{p(x_t\mid x_0)} $$ 带入并整理有 $$ p(x_{t-1}\mid x_t, x_0)=\mathcal{N}\left( x_{t-1}; \frac{\sqrt{\alpha_t(1-\bar{\alpha}_{t-1})}}{1-\bar{\alpha}_{t}}x_t+\frac{\sqrt{\bar{\alpha}_{t-1}}(1-\alpha_t)}{1-\bar{\alpha}_t}x_0, \left( \frac{\sqrt{1-\alpha_t}\sqrt{1-\bar{\alpha}_{t-1}}}{\sqrt{1-\bar{\alpha}_t}} \right)^2 \right) $$ 使用$x_0=\frac{x_t-\sqrt{1-\bar{\alpha}_t}\epsilon}{\sqrt{\bar{\alpha}_t}}$替换到公式中的$x_0$可得 $$ \begin{eqnarray} p(x_{t-1}\mid x_t, x_0) &=&\mathcal{N}\left( x_{t-1}; \frac{\sqrt{\alpha_t(1-\bar{\alpha}_{t-1})}}{1-\bar{\alpha}_{t}}x_t+\frac{\sqrt{\bar{\alpha}_{t-1}}(1-\alpha_t)}{1-\bar{\alpha}_t} \times \frac{x_t-\sqrt{1-\bar{\alpha}_t}\epsilon}{\sqrt{\bar{\alpha}_t}}, \left( \frac{\sqrt{1-\alpha_t}\sqrt{1-\bar{\alpha}_{t-1}}}{\sqrt{1-\bar{\alpha}_t}} \right)^2 \right)\\ \end{eqnarray} $$ 其中$\epsilon$为Unet识别的向神经网络中添加的噪声 ##### `q_mean_variance` 方法 扩散过程$q(x_t\mid x_{0})$的参数可以通过如下方式计算: $$ x_t \sim q(x_t\mid x_{0})=\mathcal{N}(x_t; \sqrt{\bar{\alpha_t}}x_{0}, (1-\bar{\alpha_t})I) $$ - 均值$\mu$: $\sqrt{\alpha_t}x_0$ - 方差$\sigma^2$: $1-\bar{\alpha_t}$ - 对数方差$log(\sigma^2)$: $log(1-\bar{\alpha_t})$ 注释代码如下: ```python def q_mean_variance(self, x_start, t): """用于计算扩散过程中的分布x_t ~ q(x_t | x_0)的均值和方差\n x_t ~ q(x_t | x_0)=N(x_t; sqrt_alphas_cumprod_t * x_0, (1 - alphas_cumprod_t)I) Args: x_start: 一个形状为 [N x C x ...] 的张量,表示无噪声输入数据 t: 扩散步骤数(从 0 开始计数) Returns: 均值,方差,对数方差 """ mean = (extract_into_tensor(self.sqrt_alphas_cumprod, t, x_start.shape) * x_start) variance = extract_into_tensor(1.0 - self.alphas_cumprod, t, x_start.shape) log_variance = extract_into_tensor(self.log_one_minus_alphas_cumprod, t, x_start.shape) return mean, variance, log_variance ``` ##### `predict_start_from_noise` 方法 从噪声推导原图可以通过以下公式计算: $$ x_0=\frac{x_t-\sqrt{1-\bar{\alpha}_t}\epsilon}{\sqrt{\bar{\alpha}_t}} $$ 其中$\epsilon$为模型预测的噪声 注释代码如下: ```python def predict_start_from_noise(self, x_t, t, noise): """从扩散过程某个时间步t的图像x_t和噪声ε逆推原始图像x_0 Args: x_t: 扩散过程在时间步t 时的图像。 t: 扩散的时间步索引 noise: 噪声ε Returns: 返回推导得到的原始图像 """ return ( extract_into_tensor(self.sqrt_recip_alphas_cumprod, t, x_t.shape) * x_t - extract_into_tensor(self.sqrt_recipm1_alphas_cumprod, t, x_t.shape) * noise ) ``` ##### `q_posterior` 方法 从$x_{t}$逆向推导$x_{t-1}$的公式如下: $$ \begin{eqnarray} p(x_{t-1}\mid x_t, x_0) &=&\mathcal{N}\left( x_{t-1}; \frac{\sqrt{\alpha_t(1-\bar{\alpha}_{t-1})}}{1-\bar{\alpha}_{t}}x_t+\frac{\sqrt{\bar{\alpha}_{t-1}}(1-\alpha_t)}{1-\bar{\alpha}_t} \times x_0, \left( \frac{\sqrt{1-\alpha_t}\sqrt{1-\bar{\alpha}_{t-1}}}{\sqrt{1-\bar{\alpha}_t}} \right)^2 \right)\\ \end{eqnarray} $$ - 均值$\mu=\frac{\sqrt{\alpha_t(1-\bar{\alpha}_{t-1})}}{1-\bar{\alpha}_{t}}x_t+\frac{\sqrt{\bar{\alpha}_{t-1}}(1-\alpha_t)}{1-\bar{\alpha}_t}\times x_0$ - 方差$\sigma^2=\left( \frac{\sqrt{1-\alpha_t}\sqrt{1-\bar{\alpha}_{t-1}}}{\sqrt{1-\bar{\alpha}_t}} \right)^2$ - 对数方差$log(\sigma^2)=max(1e-20, \sigma^2)$ 注释代码如下: ```python def q_posterior(self, x_start, x_t, t): """函数计算的是在时间步t时,给定初始图象x_t和扩散过程的图像x_t,逆向扩散过程q(x_{t-1}|x_t, x_0)的后验分布的均值和方差 Args: x_start: 扩散过程的初始图像 x_t: 扩散过程中时间步t的图像 t: 当前的时间步索引 Returns: 均值, 方差, 对数方差(裁剪处理后,避免方差过小不稳定) """ posterior_mean = ( extract_into_tensor(self.posterior_mean_coef1, t, x_t.shape) * x_start + extract_into_tensor(self.posterior_mean_coef2, t, x_t.shape) * x_t ) posterior_variance = extract_into_tensor(self.posterior_variance, t, x_t.shape) posterior_log_variance_clipped = extract_into_tensor(self.posterior_log_variance_clipped, t, x_t.shape) return posterior_mean, posterior_variance, posterior_log_variance_clipped ``` ##### `p_mean_variance` 方法 函数将当前时间步的图像数据和时间信息送入模型,得到预测的噪声;在根据预测得到的噪声预测初始图象,并借助初始图象来预测$q(x_{t-1}\mid x_t, x_0)$的均值方差和对数方差 注释代码如下: ```python def p_mean_variance(self, x, t, clip_denoised: bool): """计算并返回模型的均值、后验方差和后验对数方差 Args: x: 当前时间步的图像数据 t: 时间步 clip_denoised: 布尔值,指示是否将去噪后的结果裁剪到一个指定的范围内 Returns: _description_ """ model_out = self.model(x, t) # 预测得到的噪声 if self.parameterization == "eps": x_recon = self.predict_start_from_noise(x, t=t, noise=model_out) # 直接从噪声预测初始图像 elif self.parameterization == "x0": x_recon = model_out # 预测原图像 if clip_denoised: x_recon.clamp_(-1., 1.) model_mean, posterior_variance, posterior_log_variance = self.q_posterior(x_start=x_recon, x_t=x, t=t) return model_mean, posterior_variance, posterior_log_variance ``` ##### `p_sample` 方法 函数通过给定的图像信息和时间步,计算$p(x_{t-1}|x_t, x_0)$,并据此预测x_1步的图像信息 详细注释代码 ```python def p_sample(self, x, t, clip_denoised=True, repeat_noise=False): """函数通过给定的图像信息和时间步,计算p(x_{t-1}|x_t, x_0),并据此预测x_1步的图像信息 Args: x: 输入图像或特征图 t: 当前时间步或噪声水平 clip_denoised: 是否在去噪后裁剪图像. Defaults to True. 是否重复使用相同的噪声: _description_. Defaults to False. Returns: 返回x_{t-1}去噪的图像 """ b, *_, device = *x.shape, x.device model_mean, _, model_log_variance = self.p_mean_variance(x=x, t=t, clip_denoised=clip_denoised) noise = noise_like(x.shape, device, repeat_noise) # 从标准正态分布采样 # no noise when t == 0 nonzero_mask = (1 - (t == 0).float()).reshape(b, *((1,) * (len(x.shape) - 1))) # 确保时间步为0的时候不引入噪声 return model_mean + nonzero_mask * (0.5 * model_log_variance).exp() * noise ``` ##### `p_sample_loop` 方法 用于在扩散模型中进行逐步采样,逐渐将噪声图像还原为清晰的图像,同时还会根据`return_intermediates`参数决定是否返回中间的预测结果 详细代码: ```python def p_sample_loop(self, shape, return_intermediates=False): """用于在扩散模型中进行逐步采样,逐渐将噪声图像还原为清晰的图像 Args: shape: 生成的图像的形状,[b, c, h, w] return_intermediates: 指示是否返回每个时间步的中间结果. Defaults to False. Returns: 预测的x_0图像信息 """ device = self.betas.device b = shape[0] img = torch.randn(shape, device=device) # 生成初始噪声图像 intermediates = [img] for i in tqdm(reversed(range(0, self.num_timesteps)), desc='Sampling t', total=self.num_timesteps): img = self.p_sample(img, torch.full((b,), i, device=device, dtype=torch.long), clip_denoised=self.clip_denoised) if i % self.log_every_t == 0 or i == self.num_timesteps - 1: intermediates.append(img) if return_intermediates: return img, intermediates return img ``` ##### `q_sample` 方法 函数实现了从$x_0$添加噪声直接得到$x_t$,参考公式如下: $$ x_t=\sqrt{\bar{\alpha_t}}x_{0}+\sqrt{1-\bar{\alpha_t}}\bar{\epsilon_t},\quad \mathcal{N}\sim (0, I) $$ ```python def q_sample(self, x_start, t, noise=None): """从x_0添加噪声得到x_t Args: x_start: 初始的无噪声图像 t: 时间步或噪声水平 noise: 噪声张量. Defaults to None. Returns: _description_ """ noise = default(noise, lambda: torch.randn_like(x_start)) # 如果没有传递噪声张量,则初始化为与x_start同形状的高斯噪声 return (extract_into_tensor(self.sqrt_alphas_cumprod, t, x_start.shape) * x_start + extract_into_tensor(self.sqrt_one_minus_alphas_cumprod, t, x_start.shape) * noise) ``` ##### `get_loss`方法 计算预测值和目标值之间的损失,根据`self.loss_type`选择计算l1损失还是l2损失,并返回最终的损失值 ```python def get_loss(self, pred, target, mean=True): """计算预测值和目标值之间的损失 Args: pred: 模型的预测输出 target: 真实图像 mean: 是否对损失值进行平均并返回标量损失. Defaults to True. Returns: 损失值 """ if self.loss_type == 'l1': loss = (target - pred).abs() # l1损失 if mean: loss = loss.mean() elif self.loss_type == 'l2': if mean: loss = torch.nn.functional.mse_loss(target, pred) # l2损失 else: loss = torch.nn.functional.mse_loss(target, pred, reduction='none') else: raise NotImplementedError("unknown loss type '{loss_type}'") return loss ``` ##### `p_losses` 方法 这个函数用于计算真实噪声和预测噪声之间的差值 ```python def p_losses(self, x_start, t, noise=None): """计算真实噪声和预测噪声之间的差值 Args: x_start: 输入图像 t: 最大时间步 noise: 噪声张量. Defaults to None. Returns: 噪声重建损失+变分损失 """ noise = default(noise, lambda: torch.randn_like(x_start)) x_noisy = self.q_sample(x_start=x_start, t=t, noise=noise) # 加噪后的图像 model_out = self.model(x_noisy, t) loss_dict = {} if self.parameterization == "eps": target = noise elif self.parameterization == "x0": target = x_start else: raise NotImplementedError(f"Paramterization {self.parameterization} not yet supported") loss = self.get_loss(model_out, target, mean=False).mean(dim=[1, 2, 3]) log_prefix = 'train' if self.training else 'val' loss_dict.update({f'{log_prefix}/loss_simple': loss.mean()}) loss_simple = loss.mean() * self.l_simple_weight loss_vlb = (self.lvlb_weights[t] * loss).mean() loss_dict.update({f'{log_prefix}/loss_vlb': loss_vlb}) loss = loss_simple + self.original_elbo_weight * loss_vlb loss_dict.update({f'{log_prefix}/loss': loss}) return loss, loss_dict ``` ##### `forward`方法 ```python def forward(self, x, *args, **kwargs): """ddpm显示随即生成了batch_size大小的从0到num_timesteps值不等的时间步,并在每个时间步上计算损失 Args: x: 输入真实图像 Returns: 总损失, 日志信息 """ # b, c, h, w, device, img_size, = *x.shape, x.device, self.image_size # assert h == img_size and w == img_size, f'height and width of image must be {img_size}' t = torch.randint(0, self.num_timesteps, (x.shape[0],), device=self.device).long() return self.p_losses(x, t, *args, **kwargs) ``` ##### `shared_step` 方法 ```python def shared_step(self, batch): """函数从输入batch中取得图像信息,并通过前向传播计算损失和损失日志 Args: batch: 一批量的数据 Returns: 损失,损失日志 """ x = self.get_input(batch, self.first_stage_key) loss, loss_dict = self(x) return loss, loss_dict ``` ##### `training_step` 方法 ```python def training_step(self, batch, batch_idx): """执行训练步骤并返回损失 Args: batch: 当前批次的数据 batch_idx: 当前批次的索引 Returns: 总损失 """ loss, loss_dict = self.shared_step(batch) # 获得损失和损失日志 self.log_dict(loss_dict, prog_bar=True, logger=True, on_step=True, on_epoch=True) self.log("global_step", self.global_step, prog_bar=True, logger=True, on_step=True, on_epoch=False) if self.use_scheduler: # 使用调度器监控修改学习率 lr = self.optimizers().param_groups[0]['lr'] self.log('lr_abs', lr, prog_bar=True, logger=True, on_step=True, on_epoch=False) return loss # 返回损失 ``` #### `UNetModel`类 对于`UnetModel`类,重点关注模型的各个部分的构成,这里不给出具体的代码分析 ##### 时间嵌入 时间嵌入的模型结构如下图所示  ##### ResBlock `ResBlock`的模型结构如下图所示 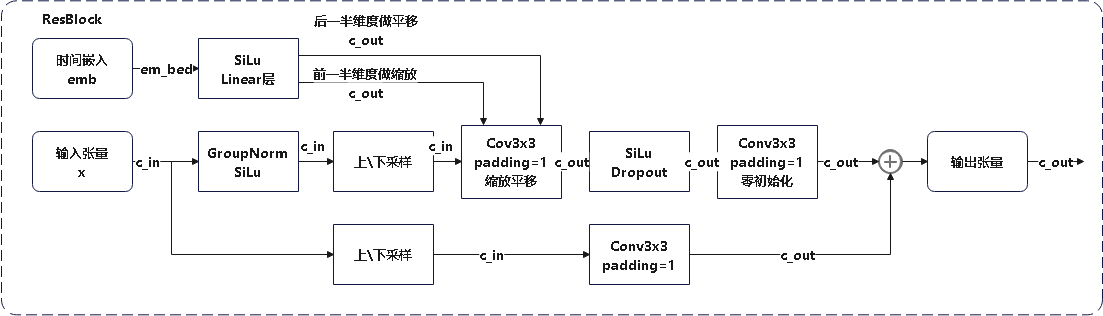 其中,当不使用`上\下采样`时,此处的模块会被一个`torch.nn.Identity`替代 ##### AttentionBlock `AttentionBlock`类的模型结构如下: 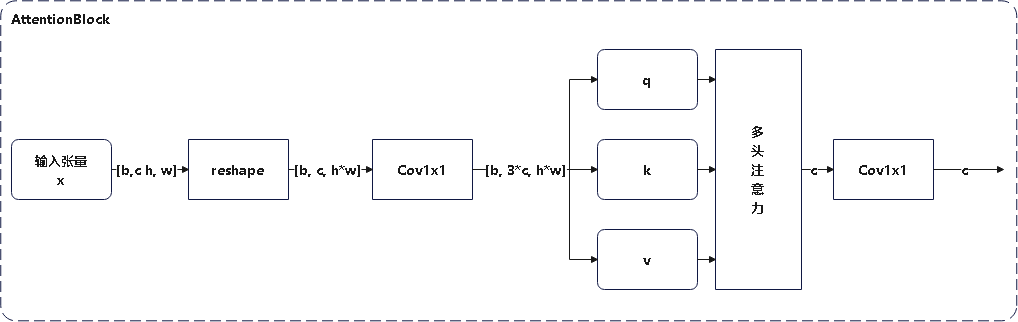 ##### 输出层 `输出层`的模型结构如下图所示  ##### 下采样层 下采样层的模型结构如下图所示: 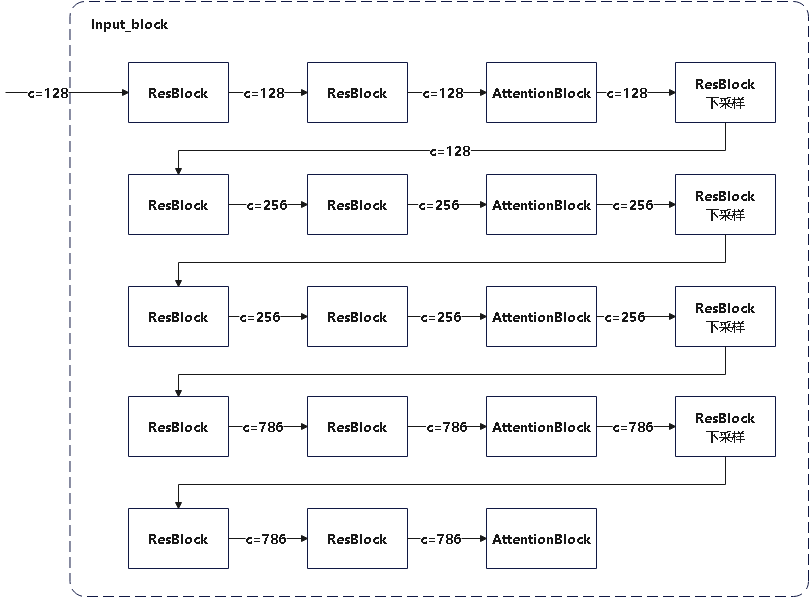 ##### 中间层 中间层的模型结构如下图所示:  ##### 上采样层 上采样层的模型结构如下图所示: 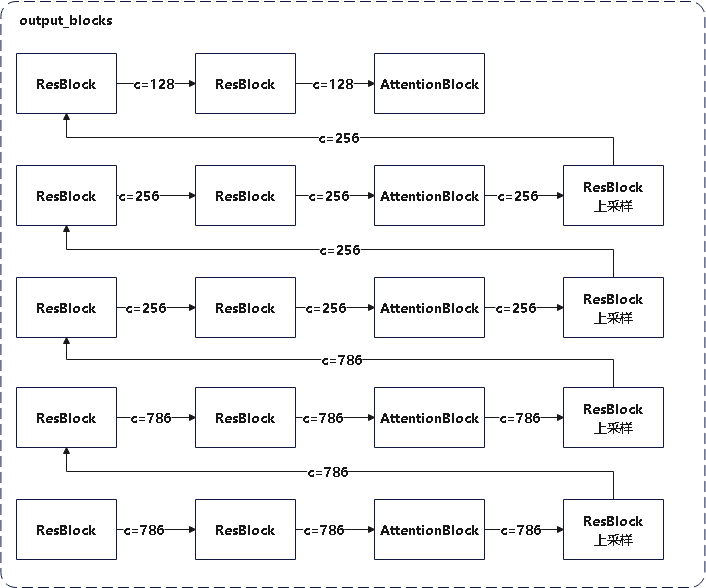 ##### 整体模型结构 整体模型结构如下: 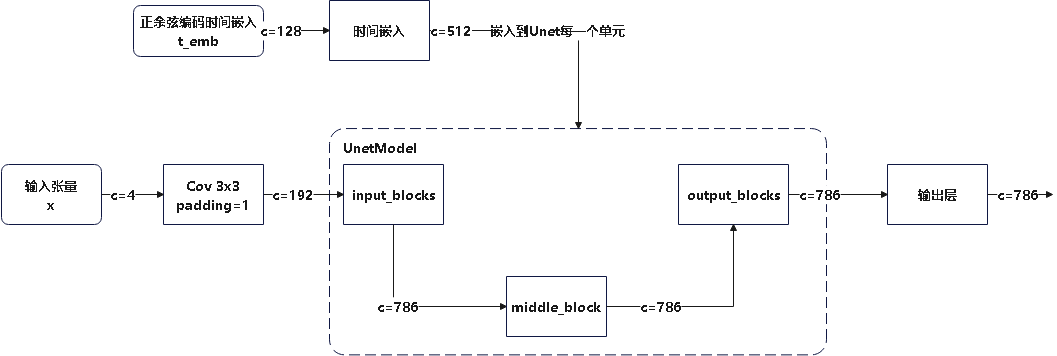 #### `LatentDiffusion` 类 > 这个类的大部分方法都类似于DDPM类,因此不详细解释 ##### `instantiate_first_stage` 方法 该函数用于从配置文件中实例化第一阶段的模型并冻结模型参数 ```python def instantiate_first_stage(self, config): """用于根据给定的配置实例化第一阶段模型 Args: config: 配置信息 """ model = instantiate_from_config(config) self.first_stage_model = model.eval() # 设置为评估模式 self.first_stage_model.train = disabled_train # 禁用模型训练 for param in self.first_stage_model.parameters(): # 冻结模型参数 param.requires_grad = False ``` ##### `instantiate_cond_stage`方法 该函数用于从配置文件中实例化条件生成模型并根据参数决定是否冻结模型参数 ```python def instantiate_cond_stage(self, config): """用于实例化条件生成模型 Args: config: 条件模型配置文件 """ if not self.cond_stage_trainable: # 不可训练模型会设置为评估模式并冻结参数 if config == "__is_first_stage__": # 使用第一阶段的模式作为条件模型 print("Using first stage also as cond stage.") self.cond_stage_model = self.first_stage_model elif config == "__is_unconditional__": # 不适用条件模型 print(f"Training {self.__class__.__name__} as an unconditional model.") self.cond_stage_model = None # self.be_unconditional = True else: # 从配置文件中加载条件模型 model = instantiate_from_config(config) self.cond_stage_model = model.eval() self.cond_stage_model.train = disabled_train for param in self.cond_stage_model.parameters(): param.requires_grad = False else: assert config != '__is_first_stage__' assert config != '__is_unconditional__' model = instantiate_from_config(config) self.cond_stage_model = model ``` ##### `__init__` 方法 ```python def __init__(self, first_stage_config, cond_stage_config, num_timesteps_cond=None, cond_stage_key="image", cond_stage_trainable=False, concat_mode=True, cond_stage_forward=None, conditioning_key=None, scale_factor=1.0, scale_by_std=False, *args, **kwargs): """LatentDiffusion,实现了潜在空间上的扩散模型 Args: first_stage_config: 自动编码器配置 cond_stage_config: 条件编码器配置 num_timesteps_cond: 用于控制时间步数的条件. Defaults to None. cond_stage_key: 条件阶段的输入数据类型. Defaults to "image". cond_stage_trainable: 条件阶段是是否训练. Defaults to False. concat_mode: _descri定义条件如何与输入拼接ption_. Defaults to True. cond_stage_forward: 规定条件阶段的前向传播方式. Defaults to None. conditioning_key: 指定如何进行条件处理. Defaults to None. scale_factor: 输入输出缩放因子. Defaults to 1.0. scale_by_std: 是否按照标准差缩放. Defaults to False. """ self.num_timesteps_cond = default(num_timesteps_cond, 1) # 1 self.scale_by_std = scale_by_std # true assert self.num_timesteps_cond <= kwargs['timesteps'] # for backwards compatibility after implementation of DiffusionWrapper if conditioning_key is None: conditioning_key = 'concat' if concat_mode else 'crossattn' if cond_stage_config == '__is_unconditional__': conditioning_key = None ckpt_path = kwargs.pop("ckpt_path", None) ignore_keys = kwargs.pop("ignore_keys", []) super().__init__(conditioning_key=conditioning_key, *args, **kwargs) self.concat_mode = concat_mode # false self.cond_stage_trainable = cond_stage_trainable # false self.cond_stage_key = cond_stage_key # image try: self.num_downs = len(first_stage_config.params.ddconfig.ch_mult) - 1 # 下采样层数 except: self.num_downs = 0 if not scale_by_std: self.scale_factor = scale_factor else: self.register_buffer('scale_factor', torch.tensor(scale_factor)) self.instantiate_first_stage(first_stage_config) self.instantiate_cond_stage(cond_stage_config) self.cond_stage_forward = cond_stage_forward # None self.clip_denoised = False self.bbox_tokenizer = None self.restarted_from_ckpt = False if ckpt_path is not None: self.init_from_ckpt(ckpt_path, ignore_keys) self.restarted_from_ckpt = True ``` ##### `encode_first_stage` 方法 该函数调用了`AutoencoderKL`的`encode`函数,实现了对于输入向量的编码 ```python def encode_first_stage(self, x): """调用第一阶段编码器模型 Args: x: 输入张量 Returns: 返回输入张量经编码器的结果 """ if hasattr(self, "split_input_params"): # 没有split_input_params if self.split_input_params["patch_distributed_vq"]: ks = self.split_input_params["ks"] # eg. (128, 128) stride = self.split_input_params["stride"] # eg. (64, 64) df = self.split_input_params["vqf"] self.split_input_params['original_image_size'] = x.shape[-2:] bs, nc, h, w = x.shape if ks[0] > h or ks[1] > w: ks = (min(ks[0], h), min(ks[1], w)) print("reducing Kernel") if stride[0] > h or stride[1] > w: stride = (min(stride[0], h), min(stride[1], w)) print("reducing stride") fold, unfold, normalization, weighting = self.get_fold_unfold(x, ks, stride, df=df) z = unfold(x) # (bn, nc * prod(**ks), L) # Reshape to img shape z = z.view((z.shape[0], -1, ks[0], ks[1], z.shape[-1])) # (bn, nc, ks[0], ks[1], L ) output_list = [self.first_stage_model.encode(z[:, :, :, :, i]) for i in range(z.shape[-1])] o = torch.stack(output_list, axis=-1) o = o * weighting # Reverse reshape to img shape o = o.view((o.shape[0], -1, o.shape[-1])) # (bn, nc * ks[0] * ks[1], L) # stitch crops together decoded = fold(o) decoded = decoded / normalization return decoded else: return self.first_stage_model.encode(x) else: return self.first_stage_model.encode(x) ``` ##### `get_first_stage_encoding`方法 根据encoder结果的对象类型选择合适的采样方式并缩放 ```python def get_first_stage_encoding(self, encoder_posterior): """根据encoder结果的对象类型选择合适的采样方式并缩放 Args: encoder_posterior: encoder返回的编码的潜在变量 Returns: 缩放后的采样向量 """ if isinstance(encoder_posterior, DiagonalGaussianDistribution): # 如果为高斯分布则采样 z = encoder_posterior.sample() elif isinstance(encoder_posterior, torch.Tensor): # 如果是张量则直接返回 z = encoder_posterior else: raise NotImplementedError(f"encoder_posterior of type '{type(encoder_posterior)}' not yet implemented") return self.scale_factor * z ``` ##### `on_train_batch_start` 方法 这个函数在训练的每个批次开始的时候被调用,用于根据潜在变量的维度设置缩放因子 ```python def on_train_batch_start(self, batch, batch_idx, dataloader_idx): """在训练每个批次的开始时被调用 Args: batch: 一批次的数据 batch_idx: 批次的id dataloader_idx: _description_ """ # only for very first batch if self.scale_by_std and self.current_epoch == 0 and self.global_step == 0 and batch_idx == 0 and not self.restarted_from_ckpt: # 确保以下操作只在第一个 epoch、第一个 global step、第一个 batch中执行 assert self.scale_factor == 1., 'rather not use custom rescaling and std-rescaling simultaneously' # set rescale weight to 1./std of encodings print("### USING STD-RESCALING ###") x = super().get_input(batch, self.first_stage_key) x = x.to(self.device) encoder_posterior = self.encode_first_stage(x) # 使用第一阶段编码器对数据进行编码,返回编码后的后验分布 z = self.get_first_stage_encoding(encoder_posterior).detach() # 采样后得到的潜在变量 del self.scale_factor self.register_buffer('scale_factor', 1. / z.flatten().std()) print(f"setting self.scale_factor to {self.scale_factor}") print("### USING STD-RESCALING ###") ``` ##### `_get_denoise_row_from_list` 方法 该方法用于从给定的样本列表中解码图像,并将他按照网格格式组织并可视化 ```python def _get_denoise_row_from_list(self, samples, desc='', force_no_decoder_quantization=False): """从给定的样本列表中解码图像,并将其组织为网格格式以便于可视化 Args: samples: 输入样本列表 desc: 进度条的描述. Defaults to ''. force_no_decoder_quantization: 是否强制使用量化. Defaults to False. Returns: _description_ """ denoise_row = [] for zd in tqdm(samples, desc=desc): denoise_row.append(self.decode_first_stage(zd.to(self.device), force_not_quantize=force_no_decoder_quantization)) n_imgs_per_row = len(denoise_row) denoise_row = torch.stack(denoise_row) # n_log_step, n_row, C, H, W denoise_grid = rearrange(denoise_row, 'n b c h w -> b n c h w') denoise_grid = rearrange(denoise_grid, 'b n c h w -> (b n) c h w') denoise_grid = make_grid(denoise_grid, nrow=n_imgs_per_row) return denoise_grid ``` ##### `get_input` 方法 `get_input`方法用于从给定的批量数据中提取输入,并进行条件编码,可返回的信息包括但不限于原输入、原输入`x`的潜在变量编码、潜在变量的解码结果、源条件输入、条件编码输出 ```python def get_input(self, batch, k, return_first_stage_outputs=False, force_c_encode=False, cond_key=None, return_original_cond=False, bs=None): """从给定的批量数据中提取输入,并进行条件编码 Args: batch: 输入的批量数据 k: 获取输入的关键字 return_first_stage_outputs: 是否返回第一阶段的输出. Defaults to False. force_c_encode: 强制条件编码的标志. Defaults to False. cond_key: 条件输入的关键字. Defaults to None. return_original_cond: 是否返回原始条件信息. Defaults to False. bs: 批量大小. Defaults to None. Returns: 原输入、原输入`x`的潜在变量编码、潜在变量的解码结果、源条件输入、条件编码输出 """ x = super().get_input(batch, k) if bs is not None: x = x[:bs] x = x.to(self.device) encoder_posterior = self.encode_first_stage(x) # 编码第一阶段的输入 z = self.get_first_stage_encoding(encoder_posterior).detach() # 禁用梯度计算 if self.model.conditioning_key is not None: # 检查是否有条件输入 # 提取相应的条件数据 if cond_key is None: cond_key = self.cond_stage_key if cond_key != self.first_stage_key: if cond_key in ['caption', 'coordinates_bbox']: xc = batch[cond_key] elif cond_key == 'class_label': xc = batch else: xc = super().get_input(batch, cond_key).to(self.device) else: xc = x if not self.cond_stage_trainable or force_c_encode: if isinstance(xc, dict) or isinstance(xc, list): # import pudb; pudb.set_trace() c = self.get_learned_conditioning(xc) # 获取条件编码 else: c = self.get_learned_conditioning(xc.to(self.device)) else: c = xc if bs is not None: c = c[:bs] if self.use_positional_encodings: # 添加位置编码信息 pos_x, pos_y = self.compute_latent_shifts(batch) ckey = __conditioning_keys__[self.model.conditioning_key] c = {ckey: c, 'pos_x': pos_x, 'pos_y': pos_y} else: c = None xc = None if self.use_positional_encodings: pos_x, pos_y = self.compute_latent_shifts(batch) c = {'pos_x': pos_x, 'pos_y': pos_y} out = [z, c] # 潜在变量, 条件编码信息 if return_first_stage_outputs: xrec = self.decode_first_stage(z) out.extend([x, xrec]) # 源输入, decoder解码信息 if return_original_cond: out.append(xc) # 源条件输入信息 return out ``` ##### `decode_first_stage` 方法 将编码后的表示`z`解码为图像,在不使用`split_input_params`的情况下,不需要关注`if hasattr(self, "split_input_params"):`这部分代码 ```python def decode_first_stage(self, z, predict_cids=False, force_not_quantize=False): if predict_cids: if z.dim() == 4: z = torch.argmax(z.exp(), dim=1).long() z = self.first_stage_model.quantize.get_codebook_entry(z, shape=None) z = rearrange(z, 'b h w c -> b c h w').contiguous() z = 1. / self.scale_factor * z if hasattr(self, "split_input_params"): if self.split_input_params["patch_distributed_vq"]: ks = self.split_input_params["ks"] # eg. (128, 128) stride = self.split_input_params["stride"] # eg. (64, 64) uf = self.split_input_params["vqf"] bs, nc, h, w = z.shape if ks[0] > h or ks[1] > w: ks = (min(ks[0], h), min(ks[1], w)) print("reducing Kernel") if stride[0] > h or stride[1] > w: stride = (min(stride[0], h), min(stride[1], w)) print("reducing stride") fold, unfold, normalization, weighting = self.get_fold_unfold(z, ks, stride, uf=uf) z = unfold(z) # (bn, nc * prod(**ks), L) # 1. Reshape to img shape z = z.view((z.shape[0], -1, ks[0], ks[1], z.shape[-1])) # (bn, nc, ks[0], ks[1], L ) # 2. apply model loop over last dim if isinstance(self.first_stage_model, VQModelInterface): output_list = [self.first_stage_model.decode(z[:, :, :, :, i], force_not_quantize=predict_cids or force_not_quantize) for i in range(z.shape[-1])] else: output_list = [self.first_stage_model.decode(z[:, :, :, :, i]) for i in range(z.shape[-1])] o = torch.stack(output_list, axis=-1) # # (bn, nc, ks[0], ks[1], L) o = o * weighting # Reverse 1. reshape to img shape o = o.view((o.shape[0], -1, o.shape[-1])) # (bn, nc * ks[0] * ks[1], L) # stitch crops together decoded = fold(o) decoded = decoded / normalization # norm is shape (1, 1, h, w) return decoded else: if isinstance(self.first_stage_model, VQModelInterface): return self.first_stage_model.decode(z, force_not_quantize=predict_cids or force_not_quantize) else: return self.first_stage_model.decode(z) else: if isinstance(self.first_stage_model, VQModelInterface): return self.first_stage_model.decode(z, force_not_quantize=predict_cids or force_not_quantize) else: return self.first_stage_model.decode(z) ``` ##### `encode_first_stage` 方法 该方法主要是在调用第一阶段的编码器模型得到潜在变量的后验分布,在不使用`split_input_params`的情况下,不需要关注`if hasattr(self, "split_input_params"):`这部分代码 ```python def encode_first_stage(self, x): """调用第一阶段编码器模型 Args: x: 输入张量 Returns: 返回输入张量经编码器的posterior """ if hasattr(self, "split_input_params"): if self.split_input_params["patch_distributed_vq"]: ks = self.split_input_params["ks"] # eg. (128, 128) stride = self.split_input_params["stride"] # eg. (64, 64) df = self.split_input_params["vqf"] self.split_input_params['original_image_size'] = x.shape[-2:] bs, nc, h, w = x.shape if ks[0] > h or ks[1] > w: ks = (min(ks[0], h), min(ks[1], w)) print("reducing Kernel") if stride[0] > h or stride[1] > w: stride = (min(stride[0], h), min(stride[1], w)) print("reducing stride") fold, unfold, normalization, weighting = self.get_fold_unfold(x, ks, stride, df=df) z = unfold(x) # (bn, nc * prod(**ks), L) # Reshape to img shape z = z.view((z.shape[0], -1, ks[0], ks[1], z.shape[-1])) # (bn, nc, ks[0], ks[1], L ) output_list = [self.first_stage_model.encode(z[:, :, :, :, i]) for i in range(z.shape[-1])] o = torch.stack(output_list, axis=-1) o = o * weighting # Reverse reshape to img shape o = o.view((o.shape[0], -1, o.shape[-1])) # (bn, nc * ks[0] * ks[1], L) # stitch crops together decoded = fold(o) decoded = decoded / normalization return decoded else: return self.first_stage_model.encode(x) else: return self.first_stage_model.encode(x) # posterior ``` ##### `shared_step` 方法 这个方法主要是根据潜在变量和条件编码信息,去计算给定条件`c`下的损失函数值 ```python def shared_step(self, batch, **kwargs): """根据潜在变量和条件编码信息,计算在给定条件下的损失函数数值 Args: batch: 批次号 Returns: 给定条件下的损失函数值 """ x, c = self.get_input(batch, self.first_stage_key) # 获取潜在变量z和条件编码信息 loss = self(x, c) # 调用前向传播,计算在给定条件下的损失函数值 return loss ``` ##### `apply_model`方法 这个方法主要是调用模型,得到重构后的图像,同样,在不使用`split_input_params`的情况下,不需要关注`if hasattr(self, "split_input_params"):`这部分代码 ```python def apply_model(self, x_noisy, t, cond, return_ids=False): if isinstance(cond, dict): # hybrid case, cond is exptected to be a dict pass else: if not isinstance(cond, list): cond = [cond] key = 'c_concat' if self.model.conditioning_key == 'concat' else 'c_crossattn' cond = {key: cond} if hasattr(self, "split_input_params"): assert len(cond) == 1 # todo can only deal with one conditioning atm assert not return_ids ks = self.split_input_params["ks"] # eg. (128, 128) stride = self.split_input_params["stride"] # eg. (64, 64) h, w = x_noisy.shape[-2:] fold, unfold, normalization, weighting = self.get_fold_unfold(x_noisy, ks, stride) z = unfold(x_noisy) # (bn, nc * prod(**ks), L) # Reshape to img shape z = z.view((z.shape[0], -1, ks[0], ks[1], z.shape[-1])) # (bn, nc, ks[0], ks[1], L ) z_list = [z[:, :, :, :, i] for i in range(z.shape[-1])] if self.cond_stage_key in ["image", "LR_image", "segmentation", 'bbox_img'] and self.model.conditioning_key: # todo check for completeness c_key = next(iter(cond.keys())) # get key c = next(iter(cond.values())) # get value assert (len(c) == 1) # todo extend to list with more than one elem c = c[0] # get element c = unfold(c) c = c.view((c.shape[0], -1, ks[0], ks[1], c.shape[-1])) # (bn, nc, ks[0], ks[1], L ) cond_list = [{c_key: [c[:, :, :, :, i]]} for i in range(c.shape[-1])] elif self.cond_stage_key == 'coordinates_bbox': assert 'original_image_size' in self.split_input_params, 'BoudingBoxRescaling is missing original_image_size' # assuming padding of unfold is always 0 and its dilation is always 1 n_patches_per_row = int((w - ks[0]) / stride[0] + 1) full_img_h, full_img_w = self.split_input_params['original_image_size'] # as we are operating on latents, we need the factor from the original image size to the # spatial latent size to properly rescale the crops for regenerating the bbox annotations num_downs = self.first_stage_model.encoder.num_resolutions - 1 rescale_latent = 2 ** (num_downs) # get top left postions of patches as conforming for the bbbox tokenizer, therefore we # need to rescale the tl patch coordinates to be in between (0,1) tl_patch_coordinates = [(rescale_latent * stride[0] * (patch_nr % n_patches_per_row) / full_img_w, rescale_latent * stride[1] * (patch_nr // n_patches_per_row) / full_img_h) for patch_nr in range(z.shape[-1])] # patch_limits are tl_coord, width and height coordinates as (x_tl, y_tl, h, w) patch_limits = [(x_tl, y_tl, rescale_latent * ks[0] / full_img_w, rescale_latent * ks[1] / full_img_h) for x_tl, y_tl in tl_patch_coordinates] # patch_values = [(np.arange(x_tl,min(x_tl+ks, 1.)),np.arange(y_tl,min(y_tl+ks, 1.))) for x_tl, y_tl in tl_patch_coordinates] # tokenize crop coordinates for the bounding boxes of the respective patches patch_limits_tknzd = [torch.LongTensor(self.bbox_tokenizer._crop_encoder(bbox))[None].to(self.device) for bbox in patch_limits] # list of length l with tensors of shape (1, 2) print(patch_limits_tknzd[0].shape) # cut tknzd crop position from conditioning assert isinstance(cond, dict), 'cond must be dict to be fed into model' cut_cond = cond['c_crossattn'][0][..., :-2].to(self.device) print(cut_cond.shape) adapted_cond = torch.stack([torch.cat([cut_cond, p], dim=1) for p in patch_limits_tknzd]) adapted_cond = rearrange(adapted_cond, 'l b n -> (l b) n') print(adapted_cond.shape) adapted_cond = self.get_learned_conditioning(adapted_cond) print(adapted_cond.shape) adapted_cond = rearrange(adapted_cond, '(l b) n d -> l b n d', l=z.shape[-1]) print(adapted_cond.shape) cond_list = [{'c_crossattn': [e]} for e in adapted_cond] else: cond_list = [cond for i in range(z.shape[-1])] # Todo make this more efficient # apply model by loop over crops output_list = [self.model(z_list[i], t, **cond_list[i]) for i in range(z.shape[-1])] assert not isinstance(output_list[0], tuple) # todo cant deal with multiple model outputs check this never happens o = torch.stack(output_list, axis=-1) o = o * weighting # Reverse reshape to img shape o = o.view((o.shape[0], -1, o.shape[-1])) # (bn, nc * ks[0] * ks[1], L) # stitch crops together x_recon = fold(o) / normalization else: x_recon = self.model(x_noisy, t, **cond) # 重建图像 if isinstance(x_recon, tuple) and not return_ids: # 如果重建图像为元组并没有指定 return x_recon[0] else: return x_recon ``` ##### `p_losses`方法 `p_losses`方法是`ddpm`在条件输入上的拓展,同样也是计算预测噪声和初始噪声的损失,并在对损失进行调整和与变分损失叠加作为最终的损失 ```python def p_losses(self, x_start, cond, t, noise=None): noise = default(noise, lambda: torch.randn_like(x_start)) # 初始噪声 x_noisy = self.q_sample(x_start=x_start, t=t, noise=noise) # 加噪后的图像 model_output = self.apply_model(x_noisy, t, cond) # 输出的重建图像 loss_dict = {} prefix = 'train' if self.training else 'val' if self.parameterization == "x0": target = x_start elif self.parameterization == "eps": target = noise else: raise NotImplementedError() loss_simple = self.get_loss(model_output, target, mean=False).mean([1, 2, 3]) # 计算得到的损失 loss_dict.update({f'{prefix}/loss_simple': loss_simple.mean()}) logvar_t = self.logvar[t].to(self.device) loss = loss_simple / torch.exp(logvar_t) + logvar_t # 对初始损失的调整 # loss = loss_simple / torch.exp(self.logvar) + self.logvar if self.learn_logvar: loss_dict.update({f'{prefix}/loss_gamma': loss.mean()}) loss_dict.update({'logvar': self.logvar.data.mean()}) loss = self.l_simple_weight * loss.mean() loss_vlb = self.get_loss(model_output, target, mean=False).mean(dim=(1, 2, 3)) loss_vlb = (self.lvlb_weights[t] * loss_vlb).mean() loss_dict.update({f'{prefix}/loss_vlb': loss_vlb}) loss += (self.original_elbo_weight * loss_vlb) # 添加变分损失 loss_dict.update({f'{prefix}/loss': loss}) return loss, loss_dict ``` ##### `forward`方法 `forward`方法用于获取在条件输入的情况下,输入图像的真实噪声和预测噪声之间的损失 ```python def forward(self, x, c, *args, **kwargs): t = torch.randint(0, self.num_timesteps, (x.shape[0],), device=self.device).long() if self.model.conditioning_key is not None: assert c is not None if self.cond_stage_trainable: # 获取条件编译输出 c = self.get_learned_conditioning(c) if self.shorten_cond_schedule: # TODO: drop this option tc = self.cond_ids[t].to(self.device) c = self.q_sample(x_start=c, t=tc, noise=torch.randn_like(c.float())) return self.p_losses(x, c, t, *args, **kwargs) ``` ##### `p_sample_loop`方法 > 函数`progressive_denoising`与这个方法类似,因此不再赘述 这个方法用于逐步生成图像的采样循环,实现了从纯噪声开始逐步去噪,直到生成最终的图像(与论文当中的图片最贴切的一集) ```python def p_sample_loop(self, cond, shape, return_intermediates=False, x_T=None, verbose=True, callback=None, timesteps=None, quantize_denoised=False, mask=None, x0=None, img_callback=None, start_T=None, log_every_t=None): """用于逐步生成图像的采样循环,实现了从纯噪声开始逐步去噪,直到生成最终的图像 Args: cond: 条件信息,用于指导生成图像,通常与输入图像相关联 shape: 生成图像的形状 return_intermediates: 是否返回中间的去噪结果. Defaults to False. x_T: 初始的随机噪声图像,如果为 None,则从标准正态分布中采样噪声. Defaults to None. verbose: 是否显示进度条. Defaults to True. callback: 每一步迭代时的回调函数,可用于监控生成过程. Defaults to None. timesteps: 生成过程中的时间步数。如果未指定,将使用默认的时间步数. Defaults to None. quantize_denoised: 是否对去噪后的图像进行量化. Defaults to False. mask: 可选的掩码,用于在生成时部分保留原图像. Defaults to None. x0: 在有 mask 的情况下,表示被掩盖的部分图像. Defaults to None. img_callback: _description_. Defaults to None. start_T: 开始的时间步,控制从哪一步开始生成. Defaults to None. log_every_t: 设置记录中间结果的步数间隔. Defaults to None. Returns: _description_ """ if not log_every_t: log_every_t = self.log_every_t device = self.betas.device b = shape[0] if x_T is None: img = torch.randn(shape, device=device) else: img = x_T intermediates = [img] if timesteps is None: timesteps = self.num_timesteps if start_T is not None: timesteps = min(timesteps, start_T) iterator = tqdm(reversed(range(0, timesteps)), desc='Sampling t', total=timesteps) if verbose else reversed( range(0, timesteps)) if mask is not None: assert x0 is not None assert x0.shape[2:3] == mask.shape[2:3] # spatial size has to match for i in iterator: ts = torch.full((b,), i, device=device, dtype=torch.long) if self.shorten_cond_schedule: assert self.model.conditioning_key != 'hybrid' tc = self.cond_ids[ts].to(cond.device) cond = self.q_sample(x_start=cond, t=tc, noise=torch.randn_like(cond)) img = self.p_sample(img, cond, ts, clip_denoised=self.clip_denoised, quantize_denoised=quantize_denoised) if mask is not None: img_orig = self.q_sample(x0, ts) img = img_orig * mask + (1. - mask) * img if i % log_every_t == 0 or i == timesteps - 1: intermediates.append(img) if callback: callback(i) if img_callback: img_callback(img, i) if return_intermediates: return img, intermediates return img ``` 其中在每个`iterator`中,`img`都要经过`p_sample`方法得到前一步预测的图像,逐步预测知道得到最初的初始图象$x_0$ ##### `sample`方法 这个方法是对`p_sample_loop`方法的一个细化,处理了可能的条件信息并将条件信息作为输入调用`p_sample_loop`方法完成采样过程. ```python def sample(self, cond, batch_size=16, return_intermediates=False, x_T=None, verbose=True, timesteps=None, quantize_denoised=False, mask=None, x0=None, shape=None,**kwargs): if shape is None: shape = (batch_size, self.channels, self.image_size, self.image_size) if cond is not None: if isinstance(cond, dict): cond = {key: cond[key][:batch_size] if not isinstance(cond[key], list) else list(map(lambda x: x[:batch_size], cond[key])) for key in cond} else: cond = [c[:batch_size] for c in cond] if isinstance(cond, list) else cond[:batch_size] return self.p_sample_loop(cond, shape, return_intermediates=return_intermediates, x_T=x_T, verbose=verbose, timesteps=timesteps, quantize_denoised=quantize_denoised, mask=mask, x0=x0) ``` 最后修改:2024 年 11 月 09 日 © 允许规范转载 赞 如果觉得我的文章对你有用,请随意赞赏